天氣預報我們每天都會關注,我們可以根據(jù)未來的天氣增減衣物、安排出行,每天的氣溫、風速風向、相對濕度、空氣質(zhì)量等成為關注的焦點。
本次使用python中requests和BeautifulSoup庫對中國天氣網(wǎng)當天和未來14天的數(shù)據(jù)進行爬取,保存為csv文件,之后用matplotlib、numpy、pandas對數(shù)據(jù)進行可視化處理和分析,得到溫濕度度變化曲線、空氣質(zhì)量圖、風向雷達圖等結(jié)果,為獲得未來天氣信息提供了有效方法。
1.數(shù)據(jù)獲取
請求網(wǎng)站鏈接
首先查看中國天氣網(wǎng)的網(wǎng)址:http://www.weather.com.cn/weather/101280701.shtml這里就訪問本地的天氣網(wǎng)址,如果想爬取不同的地區(qū)只需修改最后的101280701地區(qū)編號即可,前面的weather代表是7天的網(wǎng)頁,weather1d代表當天,weather15d代表未來14天。
這里就主要訪問7天和14天的中國天氣網(wǎng)。采用requests.get()方法,請求網(wǎng)頁,如果成功訪問,則得到的是網(wǎng)頁的所有字符串文本。這就是請求過程。
defgetHTMLtext(url): """請求獲得網(wǎng)頁內(nèi)容""" try: r=requests.get(url,timeout=30) r.raise_for_status() r.encoding=r.apparent_encoding print("成功訪問") returnr.text except: print("訪問錯誤") return""
提取有用信息
這里采用BeautifulSoup庫對剛剛獲取的字符串進行數(shù)據(jù)提取,首先對網(wǎng)頁進行檢查,找到需要獲取數(shù)據(jù)的標簽:
可以發(fā)現(xiàn)7天的數(shù)據(jù)信息在div標簽中并且id=“7d”,并且日期、天氣、溫度、風級等信息都在ul和li標簽中,所以我們可以使用BeautifulSoup對獲取的網(wǎng)頁文本進行查找div標簽id=“7d”,找出他包含的所有的ul和li標簽,之后提取標簽中相應的數(shù)據(jù)值,保存到對應列表中。
這里要注意一個細節(jié)就是有時日期沒有最高氣溫,對于沒有數(shù)據(jù)的情況要進行判斷和處理。另外對于一些數(shù)據(jù)保存的格式也要提前進行處理,比如溫度后面的攝氏度符號,日期數(shù)字的提取,和風級文字的提取,這需要用到字符查找及字符串切片處理。
defget_content(html): """處理得到有用信息保存數(shù)據(jù)文件""" final=[]#初始化一個列表保存數(shù)據(jù) bs=BeautifulSoup(html,"html.parser")#創(chuàng)建BeautifulSoup對象 body=bs.body data=body.find('div',{'id':'7d'})#找到div標簽且id=7d
下面爬取當天的數(shù)據(jù)
data2=body.find_all('div',{'class':'left-div'}) text=data2[2].find('script').string text=text[text.index('=')+1:-2]#移除改vardata=將其變?yōu)閖son數(shù)據(jù) jd=json.loads(text) dayone=jd['od']['od2']#找到當天的數(shù)據(jù) final_day=[]#存放當天的數(shù)據(jù) count=0 foriindayone: temp=[] ifcount<=23: temp.append(i['od21'])?????#?添加時間 temp.append(i['od22'])?????#?添加當前時刻溫度 temp.append(i['od24'])?????#?添加當前時刻風力方向 temp.append(i['od25'])?????#?添加當前時刻風級 temp.append(i['od26'])?????#?添加當前時刻降水量 temp.append(i['od27'])?????#?添加當前時刻相對濕度 temp.append(i['od28'])?????#?添加當前時刻控制質(zhì)量 #print(temp) final_day.append(temp) count?=?count?+1
下面爬取7天的數(shù)據(jù)
ul=data.find('ul')#找到所有的ul標簽 li=ul.find_all('li')#找到左右的li標簽 i=0#控制爬取的天數(shù) fordayinli:#遍歷找到的每一個li ifi7?and?i?>0: temp=[]#臨時存放每天的數(shù)據(jù) date=day.find('h1').string#得到日期 date=date[0:date.index('日')]#取出日期號 temp.append(date) inf=day.find_all('p')#找出li下面的p標簽,提取第一個p標簽的值,即天氣 temp.append(inf[0].string) tem_low=inf[1].find('i').string#找到最低氣溫 ifinf[1].find('span')isNone:#天氣預報可能沒有最高氣溫 tem_high=None else: tem_high=inf[1].find('span').string#找到最高氣溫 temp.append(tem_low[:-1]) iftem_high[-1]=='℃': temp.append(tem_high[:-1]) else: temp.append(tem_high) wind=inf[2].find_all('span')#找到風向 forjinwind: temp.append(j['title']) wind_scale=inf[2].find('i').string#找到風級 index1=wind_scale.index('級') temp.append(int(wind_scale[index1-1:index1])) final.append(temp) i=i+1 returnfinal_day,final
同樣對于/weather15d:15天的信息,也做同樣的處理,這里經(jīng)過查看后發(fā)現(xiàn)他的15天網(wǎng)頁中只有8-14天,前面的1-7天在/weather中,這里就分別訪問兩個網(wǎng)頁將爬取得到的數(shù)據(jù)進行合并得到最終14天的數(shù)據(jù)。
前面是未來14天的數(shù)據(jù)爬取過程,對于當天24小時的天氣信息數(shù)據(jù),經(jīng)過查找發(fā)現(xiàn)他是一個json數(shù)據(jù),可以通過json.loads() - 方法獲取當天的數(shù)據(jù),進而對當天的天氣信息進行提取。
保存csv文件
前面將爬取的數(shù)據(jù)添加到列表中,這里引入csv庫,利用f_csv.writerow(header)和f_csv.writerows(data)方法,分別寫入表頭和每一行的數(shù)據(jù),這里將1天和未來14天的數(shù)據(jù)分開存儲,分別保存為weather1.csv和weather14.csv,下面是他們保存的表格圖:
2.可視化分析
當天溫度變化曲線圖
采用matplotlib中plt.plot()方法繪制出一天24小時的溫度變化曲線,并用plt.text()方法點出最高溫和最低溫,并畫出平均溫度線,下圖為溫度變化曲線圖:(代碼見附錄)
分析可以發(fā)現(xiàn)這一天最高溫度為33℃,最低溫度為28℃,并且平均溫度在20.4℃左右,通過對時間分析,發(fā)現(xiàn)晝夜溫差5℃,低溫分布在凌晨,高溫分布在中午到下午的時間段。
當天相對濕度變化曲線圖
采用matplotlib中plt.plot()方法繪制出一天24小時的濕度變化曲線,并畫出平均相對濕度線,下圖為濕度變化曲線圖:(代碼見附錄)
分析可以發(fā)現(xiàn)這一天最高相對濕度為86%,最低相對濕度為58℃,并且平均相對濕度在75%左右,通過對時間分析,清晨的濕度比較大,而下午至黃昏濕度較小。
溫濕度相關性分析圖
經(jīng)過前面兩個圖的分析我們可以感覺到溫度和濕度之間是有關系的,為了更加清楚直觀地感受這種關系,使用plt.scatter()方法將溫度為橫坐標、濕度為縱坐標,每個時刻的點在圖中點出來,并且計算相關系數(shù),下圖為結(jié)果圖:
分析可以發(fā)現(xiàn)一天的溫度和濕度具有強烈的相關性,他們呈負相關,這就說明他們時間是負相關關系,并且進一步分析,當溫度較低時,空氣中水分含量較多,濕度自然較高,而溫度較高時,水分蒸發(fā),空氣就比較干燥,濕度較低,符合平時氣候現(xiàn)象。
空氣質(zhì)量指數(shù)柱狀圖
空氣質(zhì)量指數(shù)AQI是定量描述空氣質(zhì)量狀況的指數(shù),其數(shù)值越大說明空氣污染狀況越重,對人體健康的危害也就越大。
一般將空氣質(zhì)量指數(shù)分為6個等級,等級越高說明污染越嚴重,下面使用plt.bar方法對一天24小時的空氣質(zhì)量進行了柱狀圖繪制,并且根據(jù)6個等級的不同,相應的柱狀圖的顏色也從淺到深,也表明污染逐步加重,更直觀的顯示污染情況,并且也將最高和最低的空氣質(zhì)量指數(shù)標出,用虛線畫出平均的空氣質(zhì)量指數(shù),下圖是繪制結(jié)果圖:
上面這張是南方珠海的控制質(zhì)量圖,可以看出空氣質(zhì)量指數(shù)最大也是在健康范圍,說明珠??諝夥浅:茫治隹梢园l(fā)現(xiàn)這一天最高空氣質(zhì)量指數(shù)達到了35,最低則只有14,并且平均在25左右,通過時間也可以發(fā)現(xiàn),基本在清晨的時候是空氣最好的時候(4-9點),在下午是空氣污染最嚴重的時候,所以清晨一般可以去外面呼吸新鮮的空氣,那時污染最小。
而下面這個空氣質(zhì)量圖是選取的北方的一個城市,可以看到這里的環(huán)境遠遠比不上珠海。
風向風級雷達圖
統(tǒng)計一天的風力和風向,由于風力風向使用極坐標的方式展現(xiàn)較好,所以這里采用的是極坐標的方式展現(xiàn)一天的風力風向圖,將圓分為8份,每一份代表一個風向,半徑代表平均風力,并且隨著風級增高,藍色加深,最后結(jié)果如下所示:
分析可以發(fā)現(xiàn)這一天西南風最多,平均風級達到了1.75級,東北風也有小部分1.0級,其余空白方向無來風。
未來14天高低溫變化曲線圖
統(tǒng)計未來14天的高低溫度變化,并繪制出他們的變化曲線圖,分別用虛線將他們的平均氣溫線繪制出來,最后結(jié)果如下所示:
分析可以發(fā)現(xiàn)未來14天高溫平均氣溫為30.5℃,溫度還是比較高,但是未來的第8天有降溫,需要做好降溫準備,低溫前面處于平穩(wěn)趨勢,等到第8天開始下降,伴隨著高溫也下降,整體溫度下降,低溫平均在27℃左右。
未來14天風向風級雷達圖
統(tǒng)計未來14天的風向和平均風力,并和前面一樣采用極坐標形式,將圓周分為8個部分,代表8個方向,顏色越深代表風級越高,最后結(jié)果如下所示:
分析可以發(fā)現(xiàn)未來14天東南風、西南風所占主要風向,風級最高達到了5級,最低的西風平均風級也有3級。
未來14天氣候分布餅圖
統(tǒng)計未來14天的氣候,并求每個氣候的總天數(shù),最后將各個氣候的餅圖繪制出來,結(jié)果如下所示:
分析可以發(fā)現(xiàn)未來14天氣候基本是“雨”、“陰轉(zhuǎn)雨”和“陣雨”,下雨的天數(shù)較多,結(jié)合前面的氣溫分布圖可以看出在第8-9天氣溫高溫下降,可以推測當天下雨,導致氣溫下降。
3.結(jié)論
首先根據(jù)爬取的溫濕度數(shù)據(jù)進行的分析,溫度從早上低到中午高再到晚上低,濕度和溫度的趨勢相反,通過相關系數(shù)發(fā)現(xiàn)溫度和濕度有強烈的負相關關系,經(jīng)查閱資料發(fā)現(xiàn)因為隨著溫度升高水蒸汽蒸發(fā)加劇,空氣中水分降低濕度降低。當然,濕度同時受氣壓和雨水的影響,下雨濕度會明顯增高。
經(jīng)查閱資料空氣質(zhì)量不僅跟工廠、汽車等排放的煙氣、廢氣等有關,更為重要的是與氣象因素有關。由于晝夜溫差明顯變化,當?shù)孛鏈囟雀哂诟呖諟囟葧r,空氣上升,污染物易被帶到高空擴散;當?shù)孛鏈囟鹊陀谝欢ǜ叨鹊臏囟葧r,天空形成逆溫層,它像一個大蓋子一樣壓在地面上空,使地表空氣中各種污染物不易擴散。一般在晚間和清晨影響較大,而當太陽出來后,地面迅速升溫,逆溫層就會逐漸消散,于是污染空氣也就擴散了。
風是由氣壓在水平方向分布的不均勻?qū)е碌?。風受大氣環(huán)流、地形、水域等不同因素的綜合影響,表現(xiàn)形式多種多樣,如季風、地方性的海陸風、山谷風等,一天的風向也有不同的變化,根據(jù)未來14天的風向雷達圖可以發(fā)現(xiàn)未來所有風向基本都有涉及,并且沒有特別的某個風向,原因可能是近期沒有降水和氣文變化不大,導致風向也沒有太大的變化規(guī)律。
天氣是指某一個地區(qū)距離地表較近的大氣層在短時間內(nèi)的具體狀態(tài)。跟某瞬時內(nèi)大氣中各種氣象要素分布的綜合表現(xiàn)。根據(jù)未來14天的天氣和溫度變化可以大致推斷出某個時間的氣候,天氣和溫度之間也是有聯(lián)系的,
4.代碼框架
代碼主要分為weather.py:對中國天氣網(wǎng)進行爬取天氣數(shù)據(jù)并保存csv文件;data1_analysis.py:對當天的天氣信息進行可視化處理;data14_analysis.py:對未來14天的天氣信息進行可視化處理。下面是代碼的結(jié)構(gòu)圖:
附源代碼
weather.py #weather.py importrequests frombs4importBeautifulSoup importcsv importjson defgetHTMLtext(url): """請求獲得網(wǎng)頁內(nèi)容""" try: r=requests.get(url,timeout=30) r.raise_for_status() r.encoding=r.apparent_encoding print("成功訪問") returnr.text except: print("訪問錯誤") return"" defget_content(html): """處理得到有用信息保存數(shù)據(jù)文件""" final=[]#初始化一個列表保存數(shù)據(jù) bs=BeautifulSoup(html,"html.parser")#創(chuàng)建BeautifulSoup對象 body=bs.body data=body.find('div',{ 'id':'7d'})#找到div標簽且id=7d #下面爬取當天的數(shù)據(jù) data2=body.find_all('div',{ 'class':'left-div'}) text=data2[2].find('script').string text=text[text.index('=')+1:-2]#移除改vardata=將其變?yōu)閖son數(shù)據(jù) jd=json.loads(text) dayone=jd['od']['od2']#找到當天的數(shù)據(jù) final_day=[]#存放當天的數(shù)據(jù) count=0 foriindayone: temp=[] ifcount<=23: temp.append(i['od21'])#添加時間 temp.append(i['od22'])#添加當前時刻溫度 temp.append(i['od24'])#添加當前時刻風力方向 temp.append(i['od25'])#添加當前時刻風級 temp.append(i['od26'])#添加當前時刻降水量 temp.append(i['od27'])#添加當前時刻相對濕度 temp.append(i['od28'])#添加當前時刻控制質(zhì)量 #print(temp) final_day.append(temp) count=count+1 #下面爬取7天的數(shù)據(jù) ul=data.find('ul')#找到所有的ul標簽 li=ul.find_all('li')#找到左右的li標簽 i=0#控制爬取的天數(shù) fordayinli:#遍歷找到的每一個li ifi<7andi>0: temp=[]#臨時存放每天的數(shù)據(jù) date=day.find('h1').string#得到日期 date=date[0:date.index('日')]#取出日期號 temp.append(date) inf=day.find_all('p')#找出li下面的p標簽,提取第一個p標簽的值,即天氣 temp.append(inf[0].string) tem_low=inf[1].find('i').string#找到最低氣溫 ifinf[1].find('span')isNone:#天氣預報可能沒有最高氣溫 tem_high=None else: tem_high=inf[1].find('span').string#找到最高氣溫 temp.append(tem_low[:-1]) iftem_high[-1]=='℃': temp.append(tem_high[:-1]) else: temp.append(tem_high) wind=inf[2].find_all('span')#找到風向 forjinwind: temp.append(j['title']) wind_scale=inf[2].find('i').string#找到風級 index1=wind_scale.index('級') temp.append(int(wind_scale[index1-1:index1])) final.append(temp) i=i+1 returnfinal_day,final #print(final) defget_content2(html): """處理得到有用信息保存數(shù)據(jù)文件""" final=[]#初始化一個列表保存數(shù)據(jù) bs=BeautifulSoup(html,"html.parser")#創(chuàng)建BeautifulSoup對象 body=bs.body data=body.find('div',{ 'id':'15d'})#找到div標簽且id=15d ul=data.find('ul')#找到所有的ul標簽 li=ul.find_all('li')#找到左右的li標簽 final=[] i=0#控制爬取的天數(shù) fordayinli:#遍歷找到的每一個li ifi<8: temp=[]#臨時存放每天的數(shù)據(jù) date=day.find('span',{ 'class':'time'}).string#得到日期 date=date[date.index('(')+1:-2]#取出日期號 temp.append(date) weather=day.find('span',{ 'class':'wea'}).string#找到天氣 temp.append(weather) tem=day.find('span',{ 'class':'tem'}).text#找到溫度 temp.append(tem[tem.index('/')+1:-1])#找到最低氣溫 temp.append(tem[:tem.index('/')-1])#找到最高氣溫 wind=day.find('span',{ 'class':'wind'}).string#找到風向 if'轉(zhuǎn)'inwind:#如果有風向變化 temp.append(wind[:wind.index('轉(zhuǎn)')]) temp.append(wind[wind.index('轉(zhuǎn)')+1:]) else:#如果沒有風向變化,前后風向一致 temp.append(wind) temp.append(wind) wind_scale=day.find('span',{ 'class':'wind1'}).string#找到風級 index1=wind_scale.index('級') temp.append(int(wind_scale[index1-1:index1])) final.append(temp) returnfinal defwrite_to_csv(file_name,data,day=14): """保存為csv文件""" withopen(file_name,'a',errors='ignore',newline='')asf: ifday==14: header=['日期','天氣','最低氣溫','最高氣溫','風向1','風向2','風級'] else: header=['小時','溫度','風力方向','風級','降水量','相對濕度','空氣質(zhì)量'] f_csv=csv.writer(f) f_csv.writerow(header) f_csv.writerows(data) defmain(): """主函數(shù)""" print("Weathertest") #珠海 url1='http://www.weather.com.cn/weather/101280701.shtml'#7天天氣中國天氣網(wǎng) url2='http://www.weather.com.cn/weather15d/101280701.shtml'#8-15天天氣中國天氣網(wǎng) html1=getHTMLtext(url1) data1,data1_7=get_content(html1)#獲得1-7天和當天的數(shù)據(jù) html2=getHTMLtext(url2) data8_14=get_content2(html2)#獲得8-14天數(shù)據(jù) data14=data1_7+data8_14 #print(data) write_to_csv('weather14.csv',data14,14)#保存為csv文件 write_to_csv('weather1.csv',data1,1) if__name__=='__main__': main() data1\_analysis.py: #data1_analysis.py importmatplotlib.pyplotasplt importnumpyasnp importpandasaspd importmath deftem_curve(data): """溫度曲線繪制""" hour=list(data['小時']) tem=list(data['溫度']) foriinrange(0,24): ifmath.isnan(tem[i])==True: tem[i]=tem[i-1] tem_ave=sum(tem)/24#求平均溫度 tem_max=max(tem) tem_max_hour=hour[tem.index(tem_max)]#求最高溫度 tem_min=min(tem) tem_min_hour=hour[tem.index(tem_min)]#求最低溫度 x=[] y=[] foriinrange(0,24): x.append(i) y.append(tem[hour.index(i)]) plt.figure(1) plt.plot(x,y,color='red',label='溫度')#畫出溫度曲線 plt.scatter(x,y,color='red')#點出每個時刻的溫度點 plt.plot([0,24],[tem_ave,tem_ave],c='blue',linestyle='--',label='平均溫度')#畫出平均溫度虛線 plt.text(tem_max_hour+0.15,tem_max+0.15,str(tem_max),ha='center',va='bottom',fontsize=10.5)#標出最高溫度 plt.text(tem_min_hour+0.15,tem_min+0.15,str(tem_min),ha='center',va='bottom',fontsize=10.5)#標出最低溫度 plt.xticks(x) plt.legend() plt.title('一天溫度變化曲線圖') plt.xlabel('時間/h') plt.ylabel('攝氏度/℃') plt.show() defhum_curve(data): """相對濕度曲線繪制""" hour=list(data['小時']) hum=list(data['相對濕度']) foriinrange(0,24): ifmath.isnan(hum[i])==True: hum[i]=hum[i-1] hum_ave=sum(hum)/24#求平均相對濕度 hum_max=max(hum) hum_max_hour=hour[hum.index(hum_max)]#求最高相對濕度 hum_min=min(hum) hum_min_hour=hour[hum.index(hum_min)]#求最低相對濕度 x=[] y=[] foriinrange(0,24): x.append(i) y.append(hum[hour.index(i)]) plt.figure(2) plt.plot(x,y,color='blue',label='相對濕度')#畫出相對濕度曲線 plt.scatter(x,y,color='blue')#點出每個時刻的相對濕度 plt.plot([0,24],[hum_ave,hum_ave],c='red',linestyle='--',label='平均相對濕度')#畫出平均相對濕度虛線 plt.text(hum_max_hour+0.15,hum_max+0.15,str(hum_max),ha='center',va='bottom',fontsize=10.5)#標出最高相對濕度 plt.text(hum_min_hour+0.15,hum_min+0.15,str(hum_min),ha='center',va='bottom',fontsize=10.5)#標出最低相對濕度 plt.xticks(x) plt.legend() plt.title('一天相對濕度變化曲線圖') plt.xlabel('時間/h') plt.ylabel('百分比/%') plt.show() defair_curve(data): """空氣質(zhì)量曲線繪制""" hour=list(data['小時']) air=list(data['空氣質(zhì)量']) print(type(air[0])) foriinrange(0,24): ifmath.isnan(air[i])==True: air[i]=air[i-1] air_ave=sum(air)/24#求平均空氣質(zhì)量 air_max=max(air) air_max_hour=hour[air.index(air_max)]#求最高空氣質(zhì)量 air_min=min(air) air_min_hour=hour[air.index(air_min)]#求最低空氣質(zhì)量 x=[] y=[] foriinrange(0,24): x.append(i) y.append(air[hour.index(i)]) plt.figure(3) foriinrange(0,24): ify[i]<=50: plt.bar(x[i],y[i],color='lightgreen',width=0.7)#1等級 elify[i]<=100: plt.bar(x[i],y[i],color='wheat',width=0.7)#2等級 elify[i]<=150: plt.bar(x[i],y[i],color='orange',width=0.7)#3等級 elify[i]<=200: plt.bar(x[i],y[i],color='orangered',width=0.7)#4等級 elify[i]<=300: plt.bar(x[i],y[i],color='darkviolet',width=0.7)#5等級 elify[i]>300: plt.bar(x[i],y[i],color='maroon',width=0.7)#6等級 plt.plot([0,24],[air_ave,air_ave],c='black',linestyle='--')#畫出平均空氣質(zhì)量虛線 plt.text(air_max_hour+0.15,air_max+0.15,str(air_max),ha='center',va='bottom',fontsize=10.5)#標出最高空氣質(zhì)量 plt.text(air_min_hour+0.15,air_min+0.15,str(air_min),ha='center',va='bottom',fontsize=10.5)#標出最低空氣質(zhì)量 plt.xticks(x) plt.title('一天空氣質(zhì)量變化曲線圖') plt.xlabel('時間/h') plt.ylabel('空氣質(zhì)量指數(shù)AQI') plt.show() defwind_radar(data): """風向雷達圖""" wind=list(data['風力方向']) wind_speed=list(data['風級']) foriinrange(0,24): ifwind[i]=="北風": wind[i]=90 elifwind[i]=="南風": wind[i]=270 elifwind[i]=="西風": wind[i]=180 elifwind[i]=="東風": wind[i]=360 elifwind[i]=="東北風": wind[i]=45 elifwind[i]=="西北風": wind[i]=135 elifwind[i]=="西南風": wind[i]=225 elifwind[i]=="東南風": wind[i]=315 degs=np.arange(45,361,45) temp=[] fordegindegs: speed=[] #獲取wind_deg在指定范圍的風速平均值數(shù)據(jù) foriinrange(0,24): ifwind[i]==deg: speed.append(wind_speed[i]) iflen(speed)==0: temp.append(0) else: temp.append(sum(speed)/len(speed)) print(temp) N=8 theta=np.arange(0.+np.pi/8,2*np.pi+np.pi/8,2*np.pi/8) #數(shù)據(jù)極徑 radii=np.array(temp) #繪制極區(qū)圖坐標系 plt.axes(polar=True) #定義每個扇區(qū)的RGB值(R,G,B),x越大,對應的顏色越接近藍色 colors=[(1-x/max(temp),1-x/max(temp),0.6)forxinradii] plt.bar(theta,radii,width=(2*np.pi/N),bottom=0.0,color=colors) plt.title('一天風級圖',x=0.2,fontsize=20) plt.show() defcalc_corr(a,b): """計算相關系數(shù)""" a_avg=sum(a)/len(a) b_avg=sum(b)/len(b) cov_ab=sum([(x-a_avg)*(y-b_avg)forx,yinzip(a,b)]) sq=math.sqrt(sum([(x-a_avg)**2forxina])*sum([(x-b_avg)**2forxinb])) corr_factor=cov_ab/sq returncorr_factor defcorr_tem_hum(data): """溫濕度相關性分析""" tem=data['溫度'] hum=data['相對濕度'] plt.scatter(tem,hum,color='blue') plt.title("溫濕度相關性分析圖") plt.xlabel("溫度/℃") plt.ylabel("相對濕度/%") plt.text(20,40,"相關系數(shù)為:"+str(calc_corr(tem,hum)),fontdict={ 'size':'10','color':'red'}) plt.show() print("相關系數(shù)為:"+str(calc_corr(tem,hum))) defmain(): plt.rcParams['font.sans-serif']=['SimHei']#解決中文顯示問題 plt.rcParams['axes.unicode_minus']=False#解決負號顯示問題 data1=pd.read_csv('weather1.csv',encoding='gb2312') print(data1) tem_curve(data1) hum_curve(data1) air_curve(data1) wind_radar(data1) corr_tem_hum(data1) if__name__=='__main__': main() data14\_analysis.py: #data14_analysis.py importmatplotlib.pyplotasplt importnumpyasnp importpandasaspd importmath deftem_curve(data): """溫度曲線繪制""" date=list(data['日期']) tem_low=list(data['最低氣溫']) tem_high=list(data['最高氣溫']) foriinrange(0,14): ifmath.isnan(tem_low[i])==True: tem_low[i]=tem_low[i-1] ifmath.isnan(tem_high[i])==True: tem_high[i]=tem_high[i-1] tem_high_ave=sum(tem_high)/14#求平均高溫 tem_low_ave=sum(tem_low)/14#求平均低溫 tem_max=max(tem_high) tem_max_date=tem_high.index(tem_max)#求最高溫度 tem_min=min(tem_low) tem_min_date=tem_low.index(tem_min)#求最低溫度 x=range(1,15) plt.figure(1) plt.plot(x,tem_high,color='red',label='高溫')#畫出高溫度曲線 plt.scatter(x,tem_high,color='red')#點出每個時刻的溫度點 plt.plot(x,tem_low,color='blue',label='低溫')#畫出低溫度曲線 plt.scatter(x,tem_low,color='blue')#點出每個時刻的溫度點 plt.plot([1,15],[tem_high_ave,tem_high_ave],c='black',linestyle='--')#畫出平均溫度虛線 plt.plot([1,15],[tem_low_ave,tem_low_ave],c='black',linestyle='--')#畫出平均溫度虛線 plt.legend() plt.text(tem_max_date+0.15,tem_max+0.15,str(tem_max),ha='center',va='bottom',fontsize=10.5)#標出最高溫度 plt.text(tem_min_date+0.15,tem_min+0.15,str(tem_min),ha='center',va='bottom',fontsize=10.5)#標出最低溫度 plt.xticks(x) plt.title('未來14天高溫低溫變化曲線圖') plt.xlabel('未來天數(shù)/天') plt.ylabel('攝氏度/℃') plt.show() defchange_wind(wind): """改變風向""" foriinrange(0,14): ifwind[i]=="北風": wind[i]=90 elifwind[i]=="南風": wind[i]=270 elifwind[i]=="西風": wind[i]=180 elifwind[i]=="東風": wind[i]=360 elifwind[i]=="東北風": wind[i]=45 elifwind[i]=="西北風": wind[i]=135 elifwind[i]=="西南風": wind[i]=225 elifwind[i]=="東南風": wind[i]=315 returnwind defwind_radar(data): """風向雷達圖""" wind1=list(data['風向1']) wind2=list(data['風向2']) wind_speed=list(data['風級']) wind1=change_wind(wind1) wind2=change_wind(wind2) degs=np.arange(45,361,45) temp=[] fordegindegs: speed=[] #獲取wind_deg在指定范圍的風速平均值數(shù)據(jù) foriinrange(0,14): ifwind1[i]==deg: speed.append(wind_speed[i]) ifwind2[i]==deg: speed.append(wind_speed[i]) iflen(speed)==0: temp.append(0) else: temp.append(sum(speed)/len(speed)) print(temp) N=8 theta=np.arange(0.+np.pi/8,2*np.pi+np.pi/8,2*np.pi/8) #數(shù)據(jù)極徑 radii=np.array(temp) #繪制極區(qū)圖坐標系 plt.axes(polar=True) #定義每個扇區(qū)的RGB值(R,G,B),x越大,對應的顏色越接近藍色 colors=[(1-x/max(temp),1-x/max(temp),0.6)forxinradii] plt.bar(theta,radii,width=(2*np.pi/N),bottom=0.0,color=colors) plt.title('未來14天風級圖',x=0.2,fontsize=20) plt.show() defweather_pie(data): """繪制天氣餅圖""" weather=list(data['天氣']) dic_wea={ } foriinrange(0,14): ifweather[i]indic_wea.keys(): dic_wea[weather[i]]+=1 else: dic_wea[weather[i]]=1 print(dic_wea) explode=[0.01]*len(dic_wea.keys()) color=['lightskyblue','silver','yellow','salmon','grey','lime','gold','red','green','pink'] plt.pie(dic_wea.values(),explode=explode,labels=dic_wea.keys(),autopct='%1.1f%%',colors=color) plt.title('未來14天氣候分布餅圖') plt.show() defmain(): plt.rcParams['font.sans-serif']=['SimHei']#解決中文顯示問題 plt.rcParams['axes.unicode_minus']=False#解決負號顯示問題 data14=pd.read_csv('weather14.csv',encoding='gb2312') print(data14) tem_curve(data14) wind_radar(data14) weather_pie(data14) if__name__=='__main__': main()
-
數(shù)據(jù)
+關注
關注
8文章
7002瀏覽量
88940 -
網(wǎng)頁
+關注
關注
0文章
73瀏覽量
19314 -
python
+關注
關注
56文章
4792瀏覽量
84627
原文標題:Python 爬取天氣數(shù)據(jù)及可視化分析
文章出處:【微信號:AndroidPush,微信公眾號:Android編程精選】歡迎添加關注!文章轉(zhuǎn)載請注明出處。
發(fā)布評論請先 登錄
相關推薦
Dragonbaord 410c Python交互設計:天氣預報demo數(shù)據(jù)獲取
基于Python3對攜程網(wǎng)頁上北京五星級酒店列表的爬取
基于Python實現(xiàn)一只小爬蟲爬取拉勾網(wǎng)職位信息的方法
【HarmonyOS HiSpark Wi-Fi IoT 套件試用連載】七、獲取網(wǎng)絡天氣數(shù)據(jù)
python基礎語法及流程控制
基于Dragonbaord 410c開發(fā)板的Python交互設計(2)——如何獲取天氣數(shù)據(jù)
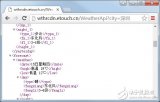
如何用python爬取抖音app數(shù)據(jù)
基于物聯(lián)網(wǎng)的天氣數(shù)據(jù)記錄器
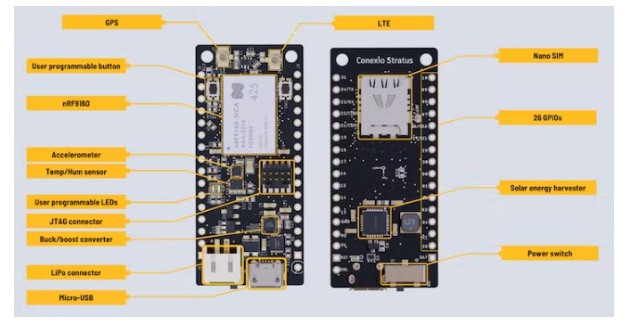
【HarmonyOS HiSpark Wi-Fi IoT 套件試用連載】七、獲取網(wǎng)絡天氣數(shù)據(jù)
天氣數(shù)據(jù)收集和存儲程序開源分享
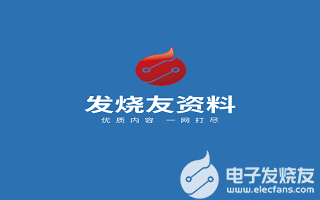
評論