作者:顏國進(jìn)英特爾邊緣計算創(chuàng)新大使
RT-DETR 是在 DETR 模型基礎(chǔ)上進(jìn)行改進(jìn)的,一種基于 DETR 架構(gòu)的實(shí)時端到端檢測器,它通過使用一系列新的技術(shù)和算法,實(shí)現(xiàn)了更高效的訓(xùn)練和推理,我們將在 Python、C++、C#三個平臺實(shí)現(xiàn) OpenVINO部署 RT-DETR 模型實(shí)現(xiàn)深度學(xué)習(xí)推理加速,在本文中,我們將首先介紹基于 OpenVINO Python API 部署 RT-DETR 模型。
該項目所使用的全部代碼已經(jīng)在 GitHub 上開源,并且收藏在 OpenVINO-CSharp-API 項目里,項目所在目錄鏈接為:
1.RT-DETR
飛槳在去年 3 月份推出了高精度通用目標(biāo)檢測模型 PP-YOLOE ,同年在 PP-YOLOE 的基礎(chǔ)上提出了 PP-YOLOE+。而繼 PP-YOLOE 提出后,MT-YOLOv6、YOLOv7、DAMO-YOLO、RTMDet 等模型先后被提出,一直迭代到今年開年的 YOLOv8。
YOLO 檢測器有個較大的待改進(jìn)點(diǎn)是需要 NMS 后處理,其通常難以優(yōu)化且不夠魯棒,因此檢測器的速度存在延遲。DETR 是一種不需要 NMS 后處理、基于 Transformer 的端到端目標(biāo)檢測器。百度飛槳正式推出了—— RT-DETR (Real-Time DEtection TRansformer),一種基于 DETR 架構(gòu)的實(shí)時端到端檢測器,其在速度和精度上取得了 SOTA 性能。
RT-DETR 是在 DETR 模型基礎(chǔ)上進(jìn)行改進(jìn)的,它通過使用一系列新的技術(shù)和算法,實(shí)現(xiàn)了更高效的訓(xùn)練和推理。具體來說,RT-DETR 具有以下優(yōu)勢:
1、實(shí)時性能更佳:RT-DETR 采用了一種新的注意力機(jī)制,能夠更好地捕獲物體之間的關(guān)系,并減少計算量。此外,RT-DETR 還引入了一種基于時間的注意力機(jī)制,能夠更好地處理視頻數(shù)據(jù)。
2、精度更高:RT-DETR 在保證實(shí)時性能的同時,還能夠保持較高的檢測精度。這主要得益于 RT-DETR 引入的一種新的多任務(wù)學(xué)習(xí)機(jī)制,能夠更好地利用訓(xùn)練數(shù)據(jù)。
3、更易于訓(xùn)練和調(diào)參:RT-DETR 采用了一種新的損失函數(shù),能夠更好地進(jìn)行訓(xùn)練和調(diào)參。此外,RT-DETR 還引入了一種新的數(shù)據(jù)增強(qiáng)技術(shù),能夠更好地利用訓(xùn)練數(shù)據(jù)。
2. OpenVINO
英特爾發(fā)行版 OpenVINO 工具套件基于 oneAPI 而開發(fā),可以加快高性能計算機(jī)視覺和深度學(xué)習(xí)視覺應(yīng)用開發(fā)速度工具套件,適用于從邊緣到云的各種英特爾平臺上,幫助用戶更快地將更準(zhǔn)確的真實(shí)世界結(jié)果部署到生產(chǎn)系統(tǒng)中。通過簡化的開發(fā)工作流程,OpenVINO 可賦能開發(fā)者在現(xiàn)實(shí)世界中部署高性能應(yīng)用程序和算法。
OpenVINO 2023.1 于 2023 年 9 月 18 日發(fā)布,該工具包帶來了挖掘生成人工智能全部潛力的新功能。生成人工智能的覆蓋范圍得到了擴(kuò)展,通過 PyTorch* 等框架增強(qiáng)了體驗,您可以在其中自動導(dǎo)入和轉(zhuǎn)換模型。大型語言模型(LLM)在運(yùn)行時性能和內(nèi)存優(yōu)化方面得到了提升。聊天機(jī)器人、代碼生成等的模型已啟用。OpenVINO 更便攜,性能更高,可以在任何需要的地方運(yùn)行:在邊緣、云中或本地。
3.環(huán)境配置
在該項目中主要包括兩個環(huán)境的配置,一個是模型的下載,另一個是模型轉(zhuǎn)換與部署,為了更好的大家復(fù)現(xiàn)該項目,所以提供主要的環(huán)境配置:
3.1 模型下載環(huán)境
paddlepaddle:2.5.1 imageio:2.31.5 imgaug:0.4.0 onnx=1.13.0 opencv-python=4.5.5.64 paddle2onnx:0.5 paddledet
左滑查看更多
3.2 模型部署環(huán)境
Numpy:1.26.0 opencv-python:4.8.1.78 openvino:2023.1.0 openvino-telemetry:2023.2.0 pillow:10.0.1 python:3.10.13
左滑查看更多
4. 模型下載與轉(zhuǎn)換
PaddleDetection提供了預(yù)訓(xùn)練模型以及模型訓(xùn)練教程,大家可以基于該教程訓(xùn)練自己的模型。在該項目中我們基于預(yù)訓(xùn)練模型展開部署案例測試,下面我們根據(jù)官方教程導(dǎo)出推理模型。
4.1 PaddlePaddle 模型下載
安裝 PaddlePaddle 和 PaddleDetection,其中 PaddlePaddle 要安裝最新版本才可以導(dǎo)出 RT-DETR 模型。安裝完成后,通過命令行下載該模型:
cd PaddleDetection python tools/export_model.py -c configs/rtdetr/rtdetr_r50vd_6x_coco.yml -o weights=https://bj.bcebos.com/v1/paddledet/models/rtdetr_r50vd_6x_coco.pdparams trt=True --output_dir=output_inference
左滑查看更多
下表為導(dǎo)出模型輸入輸出節(jié)點(diǎn)信息:
通過該表,我們可以看出,該模型存在三個輸入與兩個輸出,其中輸入“im_shape”與“scale_factor”節(jié)點(diǎn)信息主要是該模型集成了部分后處理內(nèi)容,如果大家對這種多輸入的模型使用不太習(xí)慣,在下次文章中,我們將講解如何個導(dǎo)出并部署不含后處理的模型部署流程。
因此該模型中比較關(guān)鍵的節(jié)點(diǎn)為“image”圖片數(shù)據(jù)輸入以及“reshape2_95.tmp_0”輸出節(jié)點(diǎn),其中模型輸出的格式為: [clasid, scores, x, y, w, h]。
4.2 IR 模型轉(zhuǎn)換
接下來我們將模型轉(zhuǎn)換為 IR 格式,首先將模型轉(zhuǎn) ONNX 格式:
paddle2onnx --model_dir=./output_inference/rtdetr_r50vd_6x_coco/ --model_filename model.pdmodel --params_filename model.pdiparams --opset_version 16 --save_file rtdetr_r50vd_6x_coco.onnx
左滑查看更多
由于導(dǎo)出來的模型是動態(tài)形狀,未固定 bath_size 信息,所以我們可以通過 OpenVINO 模型優(yōu)化工具對模型的輸入形狀進(jìn)行設(shè)置,命令如下:
ovc rtdetr_r50vd_6x_coco.onnx –input “ image[1,3,640,640], im_shape[1,2], scale_factor[1,2]”
左滑查看更多
最后我們可以獲取到轉(zhuǎn)憨厚的模型“ rtdetr_r50vd_6x_coco.xml”
以及“rtdetr_r50vd_6x_coco.bin”文件。
5. Python 代碼實(shí)現(xiàn)
5.1模型推理流程實(shí)現(xiàn)
在 Python 代碼中我們定義了一個 RT-DETR 模型推理方法:
def rtdert_infer(model_path, image_path, device_name, lable_path, postprocess=True):
左滑查看更多
該方法主要實(shí)現(xiàn)了 RT-DETR 模型推理全流程,包括模型讀取與加載、文件的讀取與預(yù)處理、模型推理、結(jié)果處理以及結(jié)果展示。方法輸入為:
model_path:推理模型路徑
image_path:預(yù)測圖片路徑
device_name:加速推理設(shè)備名稱
lable_path,:識別類別文件
postprocess:模型是否包含后處理,在本文中我們只講解包含后處理的模型,因此默認(rèn)為 True。
1)加載推理模型
這一步主要實(shí)現(xiàn)初始化 Core、讀取本地模型以及將模型編譯到本地,代實(shí)現(xiàn)代碼如下述代碼所示:
ie_core = Core() model = ie_core.read_model(model=model_path) compiled_model = ie_core.compile_model(model=model, device_name=device_name)
左滑查看更多
2)預(yù)處理圖片數(shù)據(jù)
這一步主要對讀取的本地圖片數(shù)據(jù)進(jìn)行處理,在此處我們定義了一個 RtdetrProcess Class 專門用于處理 RT-DETR 模型的輸入輸出數(shù)據(jù),代碼實(shí)現(xiàn)如下所示:
image = cv.imread(image_path) rtdetr_process = RtdetrProcess([640,640],lable_path) im, im_info= rtdetr_process.preprocess(image)
左滑查看更多
3)加載推理數(shù)據(jù)和模型推理
這一步主要實(shí)現(xiàn)模型推理數(shù)據(jù)的加載以及進(jìn)行模型推理,由于我們預(yù)測的模型是自帶后處理的模型,因此模型輸入有三個,分別是“im_shape”、“scale_factor”和“ image“。
im_shape:表示模型的輸入形狀,此處輸入為[640, 640];
scale_factor:表示圖片的縮放比,為模型輸入/圖像形狀;
image:表示圖像歸一化后的數(shù)據(jù)矩陣,形狀為[1, 3, 640, 640];
最后將模型輸入字典帶入到編譯好的模型中進(jìn)行模型推理,獲取推理結(jié)果。
inputs = dict() inputs["image"] = np.array(im).astype('float32') inputs["scale_factor"] = np.array(im_info['scale_factor']).reshape(1,2).astype('float32') inputs["im_shape"] = np.array([640.0,640.0]).reshape(1,2).astype('float32') results = compiled_model(inputs=inputs)
左滑查看更多
4)處理推理結(jié)果
上一步中已經(jīng)獲取到的模型推理結(jié)果,最后將模型推理結(jié)果帶入到我們定義中的后處理方法中,左后獲取模型預(yù)測結(jié)果。
re = rtdetr_process.postprocess(results[compiled_model.output(0)]) new_image=rtdetr_process.draw_box(image,re) cv.imshow("result",new_image) cv.waitKey(0)
左滑查看更多
5.2 模型數(shù)據(jù)處理方法實(shí)現(xiàn)
1)定義 RtdetrProcess
class RtdetrProcess(object): def __init__(self, target_size, label_path=None, threshold=0.5, interp=cv.INTER_LINEAR): self.im_info = dict() self.target_size =target_size self.interp = interp self.threshold = threshold if label_path is None: self.labels = [] self.flabel = False else: self.labels = self.read_lable(label_path=label_path) self.flabel = True
左滑查看更多
2)輸入數(shù)據(jù)處理方法
def preprocess(self,im): assert len(self.target_size) == 2 assert self.target_size[0] > 0 and self.target_size[1] > 0 origin_shape = im.shape[:2] resize_h, resize_w = self.target_size im_scale_y = resize_h / float(origin_shape[0]) im_scale_x = resize_w / float(origin_shape[1]) out_im = cv.cvtColor(im,cv.COLOR_BGR2RGB) out_im = cv.resize( out_im.astype('float32'), None, None, fx=im_scale_x, fy=im_scale_y, interpolation=self.interp) self.im_info['im_shape'] = np.array(im.shape[:2]).astype('float32') self.im_info['scale_factor'] = np.array([im_scale_y, im_scale_x]).astype('float32') scale = 1.0 / 255.0 out_im *= scale out_im = out_im.transpose((2, 0, 1)).copy() return np.expand_dims(out_im.astype('float32'),0), self.im_info
左滑查看更多
3)預(yù)測結(jié)果數(shù)據(jù)處理方法
def postprocess(self,scores,bboxs=None): results = [] if bboxs is None: scores = np.array(scores).astype('float32') for l in scores: if(l[1]>=self.threshold): re = dict() re["clsid"]=int(l[0]) if(self.flabel): re["label"]=self.labels[int(l[0])] else: re["label"]=int(l[0]) re["score"]=l[1] bbox=[l[2],l[3],l[4],l[5]] re["bbox"]=bbox results.append(re) else: scores = np.array(scores).astype('float32') bboxs = np.array(bboxs).astype('float32') for s,b in zip(scores,bboxs): s = self.sigmoid(s) if(np.max(np.array(s)>=self.threshold)): ids = np.argmax(np.array(s)) re = dict() re["clsid"]=int(ids) if(self.flabel): re["label"]=self.labels[int(ids)] else: re["label"]=int(ids) re["score"]=s[ids] cx=(b[0]*640.0)/self.im_info["scale_factor"][1] cy=(b[1]*640.0)/self.im_info["scale_factor"][0] w=(b[2]*640.0)/self.im_info["scale_factor"][1] h=(b[3]*640.0)/self.im_info["scale_factor"][0] bbox=[cx-w/2.0, cy-h/2.0, cx+w/2.0, cy+h/2.0] re["bbox"]=bbox results.append(re) return results
左滑查看更多
6. 預(yù)測結(jié)果展示
最后通過上述代碼,我們最終可以直接實(shí)現(xiàn) RT-DETR 模型的推理部署,RT-DETR 與訓(xùn)練模型采用的是 COCO 數(shù)據(jù)集,最終我們可以獲取預(yù)測后的圖像結(jié)果,如圖所示:
上圖中展示了 RT-DETR 模型預(yù)測結(jié)果,同時,我們對模型圖里過程中的關(guān)鍵信息以及推理結(jié)果進(jìn)行了打?。?/p>
[INFO] This is an RT-DETR model deployment case using Python! [INFO] Model path: E:Model tdetr_r50vd_6x_coco.onnx [INFO] Device name: CPU [INFO] The input path: E:GitSpaceRT-DETR-OpenVINOimage000000570688.jpg [INFO] class_id:0, label:person, confidence:0.9284, left_top:[215.03,327.88],right_bottom:[259.24,469.64] [INFO] class_id:0, label:person, confidence:0.9232, left_top:[260.34,343.99],right_bottom:[309.42,461.80] [INFO] class_id:0, label:person, confidence:0.8929, left_top:[402.26,346.80],right_bottom:[451.54,479.55] [INFO] class_id:33, label:kite, confidence:0.8382, left_top:[323.52,159.82],right_bottom:[465.93,214.78] [INFO] class_id:0, label:person, confidence:0.8342, left_top:[294.05,384.59],right_bottom:[354.15,443.96] [INFO] class_id:0, label:person, confidence:0.8284, left_top:[518.88,360.37],right_bottom:[583.88,480.00] [INFO] class_id:33, label:kite, confidence:0.8281, left_top:[282.11,217.29],right_bottom:[419.96,267.66] [INFO] class_id:33, label:kite, confidence:0.8043, left_top:[330.01,64.70],right_bottom:[389.58,86.40] [INFO] class_id:33, label:kite, confidence:0.8016, left_top:[242.46,124.74],right_bottom:[263.87,135.74] [INFO] class_id:0, label:person, confidence:0.7972, left_top:[456.74,369.06],right_bottom:[508.27,479.42] [INFO] class_id:33, label:kite, confidence:0.7970, left_top:[504.63,195.20],right_bottom:[523.44,214.82] [INFO] class_id:33, label:kite, confidence:0.7681, left_top:[460.08,251.92],right_bottom:[479.02,269.19] [INFO] class_id:33, label:kite, confidence:0.7601, left_top:[116.23,178.53],right_bottom:[137.02,190.61] [INFO] class_id:0, label:person, confidence:0.7330, left_top:[154.12,380.38],right_bottom:[210.76,421.32] [INFO] class_id:0, label:person, confidence:0.6998, left_top:[26.77,340.99],right_bottom:[58.48,425.10] [INFO] class_id:33, label:kite, confidence:0.6895, left_top:[430.29,29.91],right_bottom:[450.06,44.32] [INFO] class_id:33, label:kite, confidence:0.6739, left_top:[363.20,120.95],right_bottom:[375.84,130.11] [INFO] class_id:33, label:kite, confidence:0.6130, left_top:[176.50,236.77],right_bottom:[256.62,258.32] [INFO] class_id:0, label:person, confidence:0.6001, left_top:[497.35,380.34],right_bottom:[529.73,479.49] [INFO] class_id:33, label:kite, confidence:0.5956, left_top:[97.84,316.90],right_bottom:[156.75,360.25] [INFO] class_id:33, label:kite, confidence:0.5730, left_top:[221.56,264.66],right_bottom:[342.60,312.92] [INFO] class_id:33, label:kite, confidence:0.5555, left_top:[161.12,193.06],right_bottom:[171.45,199.78] [INFO] class_id:33, label:kite, confidence:0.5332, left_top:[171.17,317.08],right_bottom:[228.08,357.65] [INFO] class_id:33, label:kite, confidence:0.5322, left_top:[218.97,178.13],right_bottom:[451.95,241.61]
左滑查看更多
7. 總結(jié)
在本項目中,我們介紹了 OpenVINO Python API 部署自帶后處理的 RT-DETR 模型的案例,并結(jié)合該模型的處理方式封裝完整的代碼案例,實(shí)現(xiàn)了在英特爾平臺使用 OpenVINO加速深度學(xué)習(xí)模型,有助于大家以后落地 RT-DETR 模型在工業(yè)上的應(yīng)用。為了更好地大家落地 RT-DETR 模型,我們不僅開發(fā)了在 Python、C++、C#三個平臺上的案例代碼,還結(jié)合大家的模型部署習(xí)慣對該模型進(jìn)行了裁剪,實(shí)現(xiàn)了去除掉后處理的單輸入模型的部署案例。
在本文中。由于篇幅有限,對于其他編程平臺的實(shí)現(xiàn)以及不包含后處理的模型的部署案例,將在后續(xù)的文章中推出,請大家關(guān)注本平臺后續(xù)發(fā)布的文章:《基于 OpenVINO C++ API 部署 RT-DETR 模型》以及《基于 OpenVINO C#部署 RT-DETR 模型》。如果大家有興趣,可以先關(guān)注本項目代碼倉庫,獲取項目實(shí)現(xiàn)源碼。
審核編輯:湯梓紅
-
英特爾
+關(guān)注
關(guān)注
61文章
9949瀏覽量
171687 -
開源
+關(guān)注
關(guān)注
3文章
3309瀏覽量
42471 -
模型
+關(guān)注
關(guān)注
1文章
3226瀏覽量
48806 -
python
+關(guān)注
關(guān)注
56文章
4792瀏覽量
84626 -
OpenVINO
+關(guān)注
關(guān)注
0文章
92瀏覽量
196
原文標(biāo)題:基于?OpenVINO? Python API?部署?RT-DETR?模型 | 開發(fā)者實(shí)戰(zhàn)
文章出處:【微信號:英特爾物聯(lián)網(wǎng),微信公眾號:英特爾物聯(lián)網(wǎng)】歡迎添加關(guān)注!文章轉(zhuǎn)載請注明出處。
發(fā)布評論請先 登錄
相關(guān)推薦
如何使用OpenVINO C++ API部署FastSAM模型
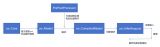
如何使用Python包裝器正確構(gòu)建OpenVINO工具套件
使用OpenVINO? 部署PaddleSeg模型庫中的DeepLabV3+模型
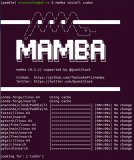
在C++中使用OpenVINO工具包部署YOLOv5模型
簡單聊聊目標(biāo)檢測新范式RT-DETR的骨干:HGNetv2
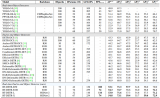
介紹RT-DETR兩種風(fēng)格的onnx格式和推理方式
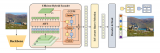
自訓(xùn)練Pytorch模型使用OpenVINO?優(yōu)化并部署在AI愛克斯開發(fā)板
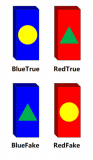
如何將Pytorch自訓(xùn)練模型變成OpenVINO IR模型形式
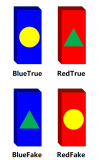
如何使用OpenVINO Python API部署FastSAM模型
NNCF壓縮與量化YOLOv8模型與OpenVINO部署測試
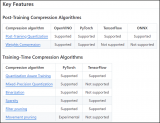
OpenVINO? Java API應(yīng)用RT-DETR做目標(biāo)檢測器實(shí)戰(zhàn)
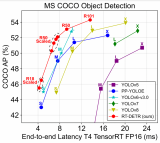
用OpenVINO C# API在intel平臺部署YOLOv10目標(biāo)檢測模型
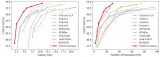
使用OpenVINO Model Server在哪吒開發(fā)板上部署模型
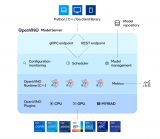
評論