筆者最近由于工作需要開始調(diào)研 Apache Doris,通過閱讀聚合函數(shù)代碼切入 Apache Doris 內(nèi)核,同時也秉承著開源的精神,開發(fā)了 array_agg 函數(shù)并貢獻(xiàn)給社區(qū)。筆者通過這篇文章記錄下對源碼的一些理解,同時也方便后面的新人更快速地上手源碼開發(fā)。
聚合函數(shù),顧名思義,即對一組數(shù)據(jù)執(zhí)行聚合計算并返回結(jié)果的函數(shù),在統(tǒng)計分析過程中屬于最常見的函數(shù)之一,最典型的聚合函數(shù)包括 count、min、max、sum 等?;诰酆虾瘮?shù)可以實現(xiàn)對大量數(shù)據(jù)的匯總計算,以更簡潔的形式呈現(xiàn)數(shù)據(jù)并支持?jǐn)?shù)據(jù)可視化。
相較于單機數(shù)據(jù)庫,由于所有數(shù)據(jù)都存儲在同一臺機器上、無需跨節(jié)點的網(wǎng)絡(luò)數(shù)據(jù)傳輸,往往單機數(shù)據(jù)庫的聚合函數(shù)執(zhí)行效率更高,而分布式數(shù)據(jù)庫由于數(shù)據(jù)分散存儲于多個節(jié)點、并行執(zhí)行計算時需要從多個節(jié)點匯集數(shù)據(jù),帶來了額外的網(wǎng)絡(luò)傳輸和本地磁盤 IO 開銷,且多副本機制和分片策略也進(jìn)一步增加了計算的數(shù)據(jù)量和管理的復(fù)雜性。
為避免單點瓶頸同時減少網(wǎng)絡(luò) IO,往往需要使用多階段的方式進(jìn)行執(zhí)行,因此 Apache Doris 實現(xiàn)了靈活的多階段聚合機制,能夠根據(jù)查詢語句的特點為其選擇適當(dāng)?shù)木酆戏绞?,從而在?zhí)行時間和執(zhí)行開銷(如內(nèi)存,IO 等)之間取得有效的平衡。
多階段聚合
在 Apache Doris 中,主要聚合機制有如下幾種:
一階段聚合:Group By 僅包含分桶列,不同 Tablet 的數(shù)據(jù)在不同的分組中,因此不同 BE 可以獨立并行計算;
兩階段聚合:Group By 包含非分桶列,同一個分組中的數(shù)據(jù)可能分布在多個 BE 上;
三階段聚合:Count Distinct 包含 Group By(即 2 個兩階段聚合的組合);
四階段聚合:Count Distinct 不包含 Group By,通常采用 4 階段聚合(1 個一階段聚合和 1 個二階段聚合的組合)
01 一階段聚合
以如下查詢?yōu)槔?,c1 是分桶列:
SELECTcount(c1)FROMt1GROUPBYc1
由于每個 BE 存儲了若干個 Tablet ,每臺 BE 只需要對當(dāng)前節(jié)點上的 Tablet Set,分別進(jìn)行 Hash Aggregate 即可,也稱為 Final Hash Aggregate,隨后對各個 BE 結(jié)果進(jìn)行匯總。
同一個 BE 可以使用多個線程來同時進(jìn)行 Final Hash Aggregate 以提高效率,這里為了便于更簡單理解僅討論單線程。
02 兩階段聚合
以如下查詢?yōu)槔?,c2 不是分桶列:
SELECTc2,count(c1)FROMt1GROUPBYc2
對于上述查詢,可以生成如下兩階段查詢:
對 scan 分區(qū)按照 group by 字段(即 c2)進(jìn)行分組聚合;
將聚合后的結(jié)果按照 group by 字段進(jìn)行重分區(qū),然后對新的分區(qū)按照 group by 字段進(jìn)行分組聚合。
具體流程如下:
BE 對本節(jié)點上的 Tablet Set 進(jìn)行第一次 Hash Aggregate,也稱為 Pre Hash Aggregate;
BE 將 Pre Hash Aggregate 產(chǎn)生的結(jié)果按照完全相同的規(guī)則進(jìn)行 Shuffle,其目的是將相同分組中的數(shù)據(jù)分發(fā)到同一臺機器上;
BE 收到 Shuffle 數(shù)據(jù)后,再次進(jìn)行 Hash Aggregate,也稱為 Final Hash Aggregate;
對各個 BE 結(jié)果進(jìn)行匯總
03 三階段聚合
以如下查詢?yōu)槔?/p>
SELECTcount(distinctc1)FROMt1GROUPBYc2
對于上述查詢,可以生成如下三階段查詢:
對 scan 分區(qū)按照 group by 和 distinct 字段(即 c2, c1)進(jìn)行分組聚合;
將聚合后的結(jié)果按照 group by 和 distinct 字段進(jìn)行重分區(qū),然后對新的分區(qū)按照 group by 和 distinct 字段進(jìn)行分組聚合;
對新的分區(qū)按照 group by 字段(即 c2)進(jìn)行分組聚合。
04 四階段聚合
以如下查詢?yōu)槔?/p>
SELECTcount(distinctc1),sum(c2)FROMt1
對于上述查詢,可以生成如下四階段查詢:
對 scan 分區(qū)按照 distinct 字段進(jìn)行分組聚合;
將聚合后的結(jié)果按照 distinct 字段進(jìn)行重分區(qū),然后對新的分區(qū)按照 distinct 字段進(jìn)行分組聚合;
將 count distinct 轉(zhuǎn)換為 count,對新的分區(qū)進(jìn)行聚合;
對各分區(qū)的結(jié)果進(jìn)行匯總聚合。
05 流式預(yù)聚合
對于上述多階段聚合中的第一階段,其主要作用是通過預(yù)聚合減少重分區(qū)產(chǎn)生的網(wǎng)絡(luò) IO。如果在聚合時使用了高基數(shù)的維度作為分組維度(如 group by ID),則預(yù)聚合的效果可能會大打折扣。為此,Apache Doris 支持為此聚合階段啟用流式預(yù)聚合,在此模式下如果 Aggregate Pipeline 發(fā)現(xiàn)聚合操作產(chǎn)生的行數(shù)減少效果不及預(yù)期,則不再對新的 Block 進(jìn)行聚合而是將其轉(zhuǎn)換后放到隊列中。而 Read Pipeline 也無需等待前者聚合完畢才開始執(zhí)行,而是讀取隊列中 Block 進(jìn)行處理,直到 Aggregate Pipeline 執(zhí)行完畢后才讀取 Hash 表中的聚合結(jié)果。
簡單而言,聚合過程中如果 Hash Table 需要擴容但發(fā)現(xiàn)聚合效果不好(比如輸入 1w 條數(shù)據(jù),經(jīng)聚合后還有 1w 個分組)就會跳過聚合,直接把每一行輸入當(dāng)作一個分組。即在第一階段,對不同的數(shù)據(jù)分布,采用不同的處理方式能夠進(jìn)一步提高效率:
若數(shù)據(jù)聚合度高,那么在該階段進(jìn)行聚合,可以有效減少數(shù)據(jù)量,降低 Shuffle 時的網(wǎng)絡(luò)開銷;
若數(shù)據(jù)聚合度低,在該階段進(jìn)行聚合無法起到很好的聚合效果,同時伴隨著額外的開銷,例如哈希計算、額外的 Map、Set 存儲空間,此時我們可以將該算子退化成一個簡單的流式傳輸?shù)乃阕?,?shù)據(jù)進(jìn)入該算子后,不做處理直接輸出。
06 Merge & Finalize
由于聚合計算的執(zhí)行過程和最終結(jié)果的生成方式不同,聚合函數(shù)可以分為需要 Finalize 的和不需要 Finalize 的這兩類。需要 Finalize 的聚合函數(shù)(在計算過程中會產(chǎn)生中間結(jié)果,這些中間結(jié)果可能需要進(jìn)一步的處理或合并才能得到最終的聚合結(jié)果)包括:
AVG:計算平均值時需要將所有值相加再除以總數(shù),因此需要 Finalize 操作來完成這個過程;
STDDEV:計算標(biāo)準(zhǔn)差時需要先計算方差再開方得到標(biāo)準(zhǔn)差,這個過程需要多次遍歷數(shù)據(jù)集,因此需要 Finalize 操作來完成;
VAR_POP、VAR_SAMP:計算方差時需要用到所有數(shù)據(jù)的平方和,這個過程需要多次遍歷數(shù)據(jù)集,因此需要 Finalize 操作來完成。
不需要 Finalize 的聚合函數(shù)(在計算過程中可以直接得到最終結(jié)果)包括:
COUNT:只需要統(tǒng)計數(shù)據(jù)集中的行數(shù),不需要進(jìn)行其他操作;
SUM、MIN、MAX:對數(shù)據(jù)集進(jìn)行聚合時,這些函數(shù)只需要遍歷一次數(shù)據(jù)集,因此不需要進(jìn)行 Finalize 操作。
對于非第一階段的聚合算子來說,由于其讀取到的是經(jīng)過聚合后的數(shù)據(jù),因此在執(zhí)行時需要將聚合狀態(tài)進(jìn)行合并。而對于最后階段的聚合算子,則需要在聚合計算后計算出最終的聚合結(jié)果。
聚合函數(shù)核心接口
01 IAggregateFunction接口
在 Apache Doris 之中,定義了一個統(tǒng)一的聚合函數(shù)接口 IAggregateFunction。上文筆者提到的聚合函數(shù),則都是作為抽象類 IAggregateFunction 的子類來實現(xiàn)的。該類中所有函數(shù)都是純虛函數(shù),需要子類自己實現(xiàn),其中該接口最為核心的方法如下:
add 函數(shù):最為核心的調(diào)用接口,將對應(yīng) AggregateDataPtr 指針之中數(shù)據(jù)取出,與列 columns 中的第 row_num 的數(shù)據(jù)進(jìn)行對應(yīng)的聚合計算。(這里可以看到 Doris 是一個純粹的列式存儲數(shù)據(jù)庫,所有的操作都是基于列的數(shù)據(jù)結(jié)構(gòu)。)
merge 函數(shù):將兩個聚合結(jié)果進(jìn)行合并的函數(shù),通常用在并發(fā)執(zhí)行聚合函數(shù)的過程之中,需要將對應(yīng)的聚合結(jié)果進(jìn)行合并。
serialize 函數(shù)與 deserialize 函數(shù):序列化與反序列化的函數(shù),通常用于 Spill-to-Disk 或 BE 節(jié)點之間傳輸中間結(jié)果的。
add_batch 函數(shù):雖然它僅僅實現(xiàn)了一個 for 循環(huán)調(diào)用 add 函數(shù),但通過這樣的方式來減少虛函數(shù)的調(diào)用次數(shù),并且增加了編譯器內(nèi)聯(lián)的概率。(虛函數(shù)的調(diào)用需要一次訪存指令,一次查表,最終才能定位到需要調(diào)用的函數(shù)上,這在傳統(tǒng)的火山模型的實現(xiàn)上會帶來極大的CPU開銷。)
首先看聚合節(jié)點 Aggregetor 是如何調(diào)用 add_batch 函數(shù):
for(inti=0;iexecute_batch_add( block,_offsets_of_aggregate_states[i],_places.data(), _agg_arena_pool.get())); }
這里依次遍歷 AggFnEvaluator 并調(diào)用 execute_batch_add-->add_batch,而 add_batch 接口就是一行行的遍歷列進(jìn)行聚合計算:
voidadd_batch(size_tbatch_size,AggregateDataPtr*places,size_tplace_offset, constIColumn**columns,Arena*arena,boolagg_many)constoverride{ for(size_ti=0;i(this)->add(places[i]+place_offset,columns,i,arena); } }
構(gòu)造函數(shù):
IAggregateFunction(constDataTypes&argument_types_): argument_types(argument_types_){}
argument_types_ 指的是函數(shù)的參數(shù)類型,比如函數(shù)select avg(a), avg(b), c from test group by c,這里 a, b 分別是 UInt16 類型與 Decimal 類型,那么這個 avg(a) 與 avg(b) 的參數(shù)就不同。
聚合函數(shù)結(jié)果輸出接口 將聚合計算的結(jié)果重新組織為列存:
///Insertsresultsintoacolumn. virtualvoidinsert_result_into(ConstAggregateDataPtr__restrictplace,IColumn&to)const=0;
首先看聚合節(jié)點 AggregationNode 是如何調(diào)用 insert_result_into 函數(shù)的: for(size_ti=0;iinsert_result_info( mapped+_offsets_of_aggregate_states[i], value_columns[i].get()); } voidAggFnEvaluator::insert_result_info(AggregateDataPtrplace,IColumn*column){ _function->insert_result_into(place,*column); }
AggregationNode 同樣是遍歷 Hash 表之中的結(jié)果,將 Key 列先組織成列存,然后調(diào)用 insert_result_info 函數(shù)將聚合計算的結(jié)果也轉(zhuǎn)換為列存。以 avg 的實現(xiàn)為例:
voidinsert_result_into(ConstAggregateDataPtr__restrictplace,IColumn&to)constoverride{ auto&column=assert_cast(to); column.get_data().push_back(this->data(place).templateresult ()); } template AggregateFunctionAvgData::ResultTresult()const{ ifconstexpr(std::is_floating_point_v ){ ifconstexpr(std::numeric_limits ::is_iec559){ returnstatic_cast (sum)/count;///allowdivisionbyzero } } //https://github.com/apache/doris/blob/master/be/src/vec/aggregate_functions/aggregate_function_avg.
這里就是調(diào)用 ConstAggregateDataPtr ,即 AggregateFunctionAvgData 的 result() 函數(shù)獲取 avg 計算的結(jié)果添加到內(nèi)存中。
02 IAggregateFunctionDataHelper 接口
這個接口是上面提到 IAggregateFunction 的輔助子類接口,主要實現(xiàn)獲取 add/serialize/deserialize 函數(shù)地址的方法。
03 抽象類 IColumn
聚合函數(shù)需要大量使用 Doris 的核心接口 IColumn 類。IColumn 接口是所有數(shù)據(jù)存儲類型的基類,其表達(dá)了所有數(shù)據(jù)的內(nèi)存結(jié)構(gòu),其他帶有具體數(shù)據(jù)類型的如:ColumnNullable、ColumnUInt8、ColumnString、ColumnVector、ColumnArray 等,都實現(xiàn)了對應(yīng)的列接口,并且在子類之中具象實現(xiàn)了不同的內(nèi)存布局。
在此以 avg 的實現(xiàn)為例(這里簡化了對 Decimal 類型的處理):
voidadd(AggregateDataPtr__restrictplace,constIColumn**columns,size_trow_num, Arena*)constoverride{ constauto&column=assert_cast(*columns[0]); this->data(place).sum+=column.get_data()[row_num].value; ++this->data(place).count; } //https://github.com/apache/doris/blob/master/be/src/vec/aggregate_functions/aggregate_function_avg.h
這里 columns 是一個二維數(shù)組,通過 columns[0] 可以取到第一列。這里只有涉及到一列,為什么 columns 是二維數(shù)組呢?因為處理多列的時候,也是通過對應(yīng)的接口,而 array 就需要應(yīng)用二維數(shù)組了。注意這里有一個強制的類型轉(zhuǎn)換,column 已經(jīng)轉(zhuǎn)換為 ColVecType 類型了,這是模板派生出 IColumn 的子類。
然后通過 IColumn 子類實現(xiàn)的 get_data() 方法獲取對應(yīng) row_num 行的數(shù)據(jù),進(jìn)行 add 函數(shù)調(diào)用就完成了一次聚合函數(shù)的計算了。由于這里是計算平均值,我們可以看到不僅僅累加了 value 還計算 count。
聚合函數(shù)主體流程
在執(zhí)行時,對應(yīng)的 Fragment 會被轉(zhuǎn)換為如下 Pipeline:
在上述 Pipeline 中,Aggregate Pipeline 負(fù)責(zé)使用 Hash 表(有 group by 的情況下)對輸入數(shù)據(jù)進(jìn)行聚合,Read Pipeline 負(fù)責(zé)讀取聚合后的數(shù)據(jù)并發(fā)送至父算子,因此兩者存在依賴關(guān)系,后者需要等待前者執(zhí)行完成后才能開始執(zhí)行。
在此僅以 BE 節(jié)點收到來自 FE 節(jié)點的 Execution Fragment 來分析。Aggregate 邏輯的入口位于 AggregationNode,處理流程根據(jù)是否啟用流式預(yù)聚合而有所不同。但是不論哪種,都依賴于 AggregationNode 的實現(xiàn)。在介紹具體實現(xiàn)之前,我們先介紹下 AggregationNode。
01 結(jié)構(gòu)體介紹
AggregationNode 的一些重要成員如下,其中中文部分是筆者添加的注釋:
classAggregationNode:public::ExecNode{ Statusinit(constTPlanNode&tnode,RuntimeState*state=nullptr)override; Statusprepare_profile(RuntimeState*state); Statusprepare(RuntimeState*state)override; //SQL中包含的聚合函數(shù)的數(shù)組 std::vector_aggregate_evaluators; //是否需要finalize,前文有提到判斷準(zhǔn)則 bool_needs_finalize; //是否需要merge bool_is_merge; //是否是第一階段聚合 bool_is_first_phase; //用來bind執(zhí)行階段需要用到的函數(shù) executor_executor; //存放聚合過程中的數(shù)據(jù) AggregatedDataVariantsUPtr_agg_data; //取出聚合結(jié)果,發(fā)送至父算子進(jìn)行處理 //進(jìn)行讀取操作,會使用get_result函數(shù)進(jìn)行處理 Statuspull(doris::RuntimeState*state,vectorized::Block*output_block,bool*eos)override; //對輸入block進(jìn)行聚合,該步驟會使用前面分配的execute函數(shù)進(jìn)行處理。 Statussink(doris::RuntimeState*state,vectorized::Block*input_block,booleos)override; //讀取聚合結(jié)果,該函數(shù)最終會調(diào)用AggregationNode::pull函數(shù)進(jìn)行讀取操作 Statusget_next(RuntimeState*state,Block*block,bool*eos)override; //執(zhí)行階段需要用到的函數(shù) Status_get_without_key_result(RuntimeState*state,Block*block,bool*eos); Status_serialize_without_key(RuntimeState*state,Block*block,bool*eos); Status_execute_without_key(Block*block); Status_merge_without_key(Block*block); Status_get_with_serialized_key_result(RuntimeState*state,Block*block,bool*eos); Status_get_result_with_serialized_key_non_spill(RuntimeState*state,Block*block,bool*eos); Status_execute_with_serialized_key(Block*block); Status_merge_with_serialized_key(Block*block); }
Apache Doris 在聚合計算過程中使用了一種比較靈活的方式,在 AggregationNode 中事先聲明了一個 executor 結(jié)構(gòu)體,其中封裝了多個 std::function,分別代表執(zhí)行階段可能需要調(diào)用到的函數(shù)。在 Prepare 階段會使用 std::bind 將函數(shù)綁定到具體的實現(xiàn)上,根據(jù)是否開啟 streaming pre-agg、是否存在 group by、是否存在 distinct 等條件來確定具體綁定什么函數(shù)。
structAggregationNode::executor{ vectorized_executeexecute; vectorized_pre_aggpre_agg; vectorized_get_resultget_result; vectorized_closerclose; vectorized_update_memusageupdate_memusage; }
這幾個函數(shù)的大致調(diào)用關(guān)系過程可如下所示:
對應(yīng)的相關(guān)綁定過程:
02 普通聚合
在沒有啟用流式預(yù)聚合的情況下,處理流程如下:
1. 調(diào)用 AggregationNode::init 函數(shù)進(jìn)行初始化,包含如下處理邏輯:
調(diào)用 VExpr::create_expr_trees 函數(shù)創(chuàng)建 group by 相關(guān)的信息;
調(diào)用 AggFnEvaluator::create 函數(shù)創(chuàng)建聚合函數(shù)。在代碼中,這里是一個 for 循環(huán),即如果 SQL 中包含多個聚合函數(shù),需要創(chuàng)建多次。
2. 調(diào)用 AggregationNode::prepare 函數(shù)執(zhí)行運行前的準(zhǔn)備,包含如下處理邏輯:
調(diào)用 ExecNode::prepare 函數(shù)為父類成員執(zhí)行運行前的準(zhǔn)備;
對 group by 表達(dá)式調(diào)用 VExpr::prepare 函數(shù)執(zhí)行運行前的準(zhǔn)備;
計算聚合函數(shù)需要的狀態(tài)空間大小及各聚合函數(shù)的偏移,這些偏移量后續(xù)取地址的時候會用到
AggregationNode::prepare_profile 根據(jù)當(dāng)前聚合類型及是否涉及 group by 參數(shù) bind 對應(yīng)的處理函數(shù),分配邏輯如下:
如果當(dāng)前聚合包含 group by 參數(shù):
如果當(dāng)前聚合需要 merge 聚合狀態(tài)(多階段聚合),則使用 AggregationNode::_merge_with_serialized_key 函數(shù)用于處理輸入 block(下稱 execute 函數(shù)),否則使用 AggregationNode::_execute_with_serialized_key 函數(shù)。如果是多階段聚合多個 AggregationNode 會分別綁定_merge_with_serialized_key 和 _execute_with_serialized_key。
如果當(dāng)前聚合需要對聚合結(jié)果執(zhí)行 finalize,則使用 AggregationNode::_get_with_serialized_key_result 函數(shù)用于讀取聚合結(jié)果(下稱 get_result 函數(shù)),否則使用AggregationNode::_serialize_with_serialized_key_result 函數(shù)。
如果當(dāng)前聚合不包含 group by 參數(shù):
如果當(dāng)前聚合需要 merge 聚合狀態(tài),則使用 AggregationNode::_merge_without_key 函數(shù)用于處理輸入 block(下稱execute函數(shù)),否則使用 AggregationNode::_execute_without_key 函數(shù)。
如果當(dāng)前聚合需要對聚合結(jié)果執(zhí)行 finalize,則使用 AggregationNode::_get_with_serialized_key_result 函數(shù)用于讀取聚合結(jié)果(下稱 get_result 函數(shù)),否則使用 AggregationNode::_serialize_with_serialized_key_result 函數(shù)。
如果當(dāng)前聚合包含 group by 參數(shù),則需要根據(jù)參數(shù)類型分配對應(yīng)的 hash 方法:_init_hash_method
3. 調(diào)用 AggregationNode::sink 函數(shù)對輸入 Block 進(jìn)行聚合,該步驟會使用前面分配的 execute 函數(shù)進(jìn)行處理。 4. 調(diào)用 AggregationNode::get_next 函數(shù)讀取聚合結(jié)果,該函數(shù)最終會調(diào)用 AggregationNode::pull 函數(shù)進(jìn)行讀取操作,后者會使用前面分配的 get_result 函數(shù)進(jìn)行處理。 5. 調(diào)用 AggregationNode::release_resource 函數(shù)釋放資源,該函數(shù)會調(diào)用 _executor.close()。
對 block 數(shù)據(jù)的聚合邏輯較為簡單,以包含 group by 參數(shù)的情況為例,聚合流程如下:
調(diào)用 AggregationNode::_emplace_into_hash_table 函數(shù)創(chuàng)建具體的聚合方法類,并獲取 Hash 表中對應(yīng)行的聚合狀態(tài)。
如果當(dāng)前聚合處理的是原始的行數(shù)據(jù),則調(diào)用 AggFnEvaluator::execute_batch_add 函數(shù)進(jìn)行聚合處理。
如果當(dāng)前聚合需要 merge 聚合狀態(tài),則首先需要對聚合狀態(tài)中的結(jié)果進(jìn)行反序列化,然后調(diào)用 IAggregateFunctionHelper::merge_vec 函數(shù)對當(dāng)前聚合狀態(tài)進(jìn)行合并。
03 流式聚合
對于 hash 分組效果不佳的場景,會啟用流式預(yù)聚合,處理流程如下:
調(diào)用 AggregationNode::init 函數(shù)進(jìn)行初始化;
調(diào)用AggregationNode::prepare函數(shù)執(zhí)行運行前的準(zhǔn)備;
調(diào)用 AggregationNode::do_pre_agg 函數(shù)對輸入 block 進(jìn)行聚合,該函數(shù)會調(diào)用 _pre_agg_with_serialized_key 函數(shù)進(jìn)行實際的聚合操作。如果在處理過程中 hash 擴容達(dá)到閾值,則跳過聚合,直接把每一行輸入當(dāng)作一個分組,即調(diào)用 streaming_agg_serialize_to_column,否則還是使用樸素的方法 AggFnEvaluator::execute_batch_add;
調(diào)用 AggregationNode::pull 函數(shù)取出聚合結(jié)果,發(fā)送至父算子進(jìn)行處理;
調(diào)用 AggregationNode::release_resource 函數(shù)釋放資源。
感興趣的讀者可以自行閱讀流式聚合相關(guān)的源碼,可以給 streaming_agg_serialize_to_column 加斷點進(jìn)行 debug,觸發(fā)方法如下:
TPC-H 準(zhǔn)備 3G 數(shù)據(jù),方法見 https://doris.apache.org/zh-CN/docs/1.2/benchmark/tpch/
執(zhí)行 SQLselect count() from (select map_agg(o_orderstatus,o_clerk) from orders group by o_custkey, o_orderdate) a
如何新增一個聚合函數(shù)
下面以 map_agg 為例介紹添加聚合函數(shù)的流程。以下內(nèi)容僅為筆者個人的思考,感興趣的讀者可以具體參考 https://github.com/apache/doris/pull/22043。
01 map_agg 使用介紹
語法:MAP_AGG(expr1, expr2)
功能:返回一個 map,由 expr1 作為鍵、expr2 作為對應(yīng)的值。
02 在 FE 創(chuàng)建函數(shù)簽名
Step 1: 維護(hù) FunctionSet.java(https://github.com/apache/doris/blob/master/fe/fe-core/src/main/java/org/apache/doris/catalog/FunctionSet.java)
FE 通過 initAggregateBuiltins 來描述聚合函數(shù),所有的聚合函數(shù)都會注冊在 FunctionSet 中。初始化階段在FunctionSet.java (https://github.com/apache/doris/blob/master/fe/fe-core/src/main/java/org/apache/doris/catalog/FunctionSet.java)的 initAggregateBuiltins 中增加對應(yīng)的 AggregateFunction.createBuiltin 函數(shù)即可。
if(!Type.JSONB.equals(t)){ for(TypevalueType:Type.getMapSubTypes()){ addBuiltin(AggregateFunction.createBuiltin(MAP_AGG,Lists.newArrayList(t,valueType), newMapType(t,valueType), Type.VARCHAR, "","","","","",null,"", true,true,false,true)); } for(Typev:Type.getArraySubTypes()){ addBuiltin(AggregateFunction.createBuiltin(MAP_AGG,Lists.newArrayList(t,newArrayType(v)), newMapType(t,newArrayType(v)), newMapType(t,newArrayType(v)), "","","","","",null,"", true,true,false,true)); } }
以上代碼的理解思路如下:
如果 map_agg 的 key 不是 josn blob 字段( if (!Type.JSONB.equals(t)) ),則先找到 map_agg 相關(guān)函數(shù) ( for (Type valueType : Type.getMapSubTypes())) 。
通過 addBuiltin 初始化對應(yīng) MAP_AGG 函數(shù),value 類型是傳進(jìn)來的 valueType,中間狀態(tài)變量是 Type.VARCHAR。
找到 array 相關(guān)函數(shù)( for (Type v : Type.getArraySubTypes())),通過 addBuiltin 初始化對應(yīng) MAP_AGG 函數(shù), value 類型是 ArrayType,中間狀態(tài)變量是 MapType。
Step 2:維護(hù) AggregateFunction.java(https://github.com/apache/doris/blob/master/fe/fe-core/src/main/java/org/apache/doris/catalog/AggregateFunction.java)
在 AggregateFunction.java (https://github.com/apache/doris/blob/master/fe/fe-core/src/main/java/org/apache/doris/catalog/AggregateFunction.java)文件中,注冊 FunctionSet.MAP_AGG,具體如下:
publicstaticImmutableSetStep 3: 維護(hù) FunctionCallExpr.java (https://github.com/apache/doris/blob/master/fe/fe-core/src/main/java/org/apache/doris/analysis/FunctionCallExpr.java) 在 FunctionCallExpr.java (https://github.com/apache/doris/blob/master/fe/fe-core/src/main/java/org/apache/doris/analysis/FunctionCallExpr.java) 中根據(jù) argumentt 強制設(shè)置類型,防止丟失 decimal 類型的 scale。NOT_NULLABLE_AGGREGATE_FUNCTION_NAME_SET=ImmutableSet.of("row_number","rank", "dense_rank","multi_distinct_count","multi_distinct_sum",FunctionSet.HLL_UNION_AGG, FunctionSet.HLL_UNION,FunctionSet.HLL_RAW_AGG,FunctionSet.BITMAP_UNION,FunctionSet.BITMAP_INTERSECT, FunctionSet.ORTHOGONAL_BITMAP_INTERSECT,FunctionSet.ORTHOGONAL_BITMAP_INTERSECT_COUNT, FunctionSet.ORTHOGONAL_BITMAP_EXPR_CALCULATE_COUNT,FunctionSet.ORTHOGONAL_BITMAP_EXPR_CALCULATE, FunctionSet.INTERSECT_COUNT,FunctionSet.ORTHOGONAL_BITMAP_UNION_COUNT, FunctionSet.COUNT,"approx_count_distinct","ndv",FunctionSet.BITMAP_UNION_INT, FunctionSet.BITMAP_UNION_COUNT,"ndv_no_finalize",FunctionSet.WINDOW_FUNNEL,FunctionSet.RETENTION, FunctionSet.SEQUENCE_MATCH,FunctionSet.SEQUENCE_COUNT,FunctionSet.MAP_AGG);
if(fnName.getFunction().equalsIgnoreCase("map_agg")){ fn.setReturnType(newMapType(getChild(0).type,getChild(1).type)); }
03 在 BE 中注冊函數(shù)
這一步是為了讓 AggregateFunctionSimpleFactory 可以根據(jù)函數(shù)名找到對應(yīng)的函數(shù),函數(shù)的創(chuàng)建通過 factory.register_function_both 實現(xiàn),相關(guān)的改動可以在 aggregate_function_map.cc (https://github.com/xingyingone/doris/blob/b41fcbb7834bf89f9744d351b1cfb9ac2485008b/be/src/vec/aggregate_functions/aggregate_function_map.cpp) 中 grep register_aggregate_function_map_agg 看到,比較簡單,在此不再贅述。
04 在 BE 實現(xiàn)函數(shù)的計算邏輯
重點是如何描述中間結(jié)果以及 AggregateFunctionMapAgg 如何實現(xiàn) IAggregateFunction的核心接口。
Step 1:轉(zhuǎn)換類型
由于我們最終結(jié)果需要返回一系列 map,所以輸出類型為 DataTypeMap:
DataTypePtrget_return_type()constoverride{ ///keysandvaluescolumnof`ColumnMap`arealwaysnullable. returnstd::make_shared(make_nullable(argument_types[0]), make_nullable(argument_types[1])); }
由于默認(rèn)的中間狀態(tài)是 string 類型,如果是 string,需要處理比較復(fù)雜的序列化/反序列化操作。
IAggregateFunction::get_serialized_type(){returnstd::make_shared();}
所以在 AggregateFunctionMapAgg 重新了序列化/反序列化的中間類型:
[[nodiscard]]MutableColumnPtrcreate_serialize_column()constoverride{ returnget_return_type()->create_column(); } [[nodiscard]]DataTypePtrget_serialized_type()constoverride{returnget_return_type();}
Step 2:聚合操作
代碼中需要將每行的數(shù)據(jù)取出來進(jìn)行對應(yīng)的聚合計算,具體是通重寫 add 函數(shù)來實現(xiàn)的:
這里表示將第 row_num 行的數(shù)據(jù)丟給 AggregateFunctionMapAggData 來執(zhí)行,這里可以看出來需要對 nullable 和非 nullable 的分開處理。
在 AggregateFunctionMapAggData 中,將 key 以及 value 分別存儲在 _key_column 和 _value_column。由于 key 不為 NULL,所以執(zhí)行了 remove_nullable;由于 value 允許為 NULL,這里執(zhí)行了 make_nullable,并通過 _map 來過濾了重復(fù)的 key。
具體的代碼實現(xiàn)如下:
voidAggregateFunctionMapAgg::add(AggregateDataPtr__restrictplace,constIColumncolumns,size_trow_num, Arena*arena)constoverride{ if(columns[0]->is_nullable()){ auto&nullable_col=assert_cast(*columns[0]); auto&nullable_map=nullable_col.get_null_map_data(); if(nullable_map[row_num]){ return; } Fieldvalue; columns[1]->get(row_num,value); this->data(place).add( assert_cast (nullable_col.get_nested_column()) .get_data_at(row_num), value); }else{ Fieldvalue; columns[1]->get(row_num,value); this->data(place).add( assert_cast (*columns[0]).get_data_at(row_num),value); } } AggregateFunctionMapAggData::add(constStringRef&key,constField&value){ DCHECK(key.data!=nullptr); if(UNLIKELY(_map.find(key)!=_map.end())){ return; } ArenaKeyHolderkey_holder{key,_arena}; if(key.size>0){ key_holder_persist_key(key_holder); } _map.emplace(key_holder.key,_key_column->size()); _key_column->insert_data(key_holder.key.data,key_holder.key.size); _value_column->insert(value); }
Step 3:序列化/反序列化
由于中間傳輸?shù)氖?ColumnMap 類型,所以只需進(jìn)行數(shù)據(jù)拷貝即可
voiddeserialize_from_column(AggregateDataPtrplaces,constIColumn&column,Arena*arena, size_tnum_rows)constoverride{ auto&col=assert_cast(column); auto*data=&(this->data(places)); for(size_ti=0;i!=num_rows;++i){ automap=doris::get
Step 4:輸出結(jié)果
insert_result_into 表示最終的返回,所以里面轉(zhuǎn)換的類型要跟 return_type 里面的一致,所以可以看到我們將類型轉(zhuǎn)換為 ColumnMap 進(jìn)行處理。
voidAggregateFunctionMapAgg::insert_result_into(ConstAggregateDataPtr__restrictplace,IColumn&to)constoverride{ this->data(place).insert_result_into(to); } voidAggregateFunctionMapAggData::insert_result_into(IColumn&to)const{ auto&dst=assert_cast(to); size_tnum_rows=_key_column->size(); auto&offsets=dst.get_offsets(); auto&dst_key_column=assert_cast (dst.get_keys()); dst_key_column.get_null_map_data().resize_fill(dst_key_column.get_null_map_data().size()+ num_rows); dst_key_column.get_nested_column().insert_range_from(*_key_column,0,num_rows); dst.get_values().insert_range_from(*_value_column,0,num_rows); if(offsets.size()==0){ offsets.push_back(num_rows); }else{ offsets.push_back(offsets.back()+num_rows); } }
Step 5:維護(hù)測試用例及文檔
這塊比較簡單,可以參考官方文檔 https://doris.apache.org/zh-CN/community/developer-guide/regression-testing/
array_agg 源碼解析
筆者通過閱讀 mag_agg (https://github.com/apache/doris/pull/22043/files) 源碼以及社區(qū)大佬 @mrhhsg 的答疑解惑,為 Apache Doris 增加了 array_agg 函數(shù)支持。下文筆者將從 SQL 執(zhí)行的角度闡述上文提到的函數(shù)執(zhí)行流程及調(diào)用棧,具體代碼可以閱讀 https://github.com/apache/doris/pull/23474/files。
01 array_agg 使用介紹
語法:ARRAY_AGG(col)
功能:將一列中的值(包括空值 null)串聯(lián)成一個數(shù)組,可以用于多行轉(zhuǎn)一行(行轉(zhuǎn)列)。
需要注意點:
數(shù)組中元素不保證順序;
返回轉(zhuǎn)換生成的數(shù)組,數(shù)組中的元素類型與 col類型一致;
需要顯示 NULL
實驗 SQL 如下:
CREATETABLE`test_array_agg`( `id`int(11)NOTNULL, `label_name`varchar(32)defaultnull, `value_field`stringdefaultnull, )ENGINE=OLAP DUPLICATEKEY(`id`) COMMENT'OLAP' DISTRIBUTEDBYHASH(`id`)BUCKETS1 PROPERTIES( "replication_allocation"="tag.location.default:1", "storage_format"="V2", "light_schema_change"="true", "disable_auto_compaction"="false", "enable_single_replica_compaction"="false" ); insertinto`test_array_agg`values (1,"alex",NULL), (1,"LB","V1_2"), (1,"LC","V1_3"), (2,"LA","V2_1"), (2,"LB","V2_2"), (2,"LC","V2_3"), (3,"LA","V3_1"), (3,NULL,NULL), (3,"LC","V3_3"), (4,"LA","V4_1"), (4,"LB","V4_2"), (4,"LC","V4_3"), (5,"LA","V5_1"), (5,"LB","V5_2"), (5,"LC","V5_3");
02 執(zhí)行流程
group by + 多階段聚合
mysql>SELECTlabel_name,array_agg(label_name)FROMtest_array_aggGROUPBYlabel_name; +------------+--------------------------------+ |label_name|array_agg(`label_name`)| +------------+--------------------------------+ |LC|["LC","LC","LC","LC","LC"]| |NULL|[NULL]| |alex|["alex"]| |LB|["LB","LB","LB","LB"]| |LA|["LA","LA","LA","LA"]| +------------+--------------------------------+ 5rowsinset(11.55sec) #執(zhí)行 AggregationNode::_pre_agg_with_serialized_key-->add(執(zhí)行15次,每次處理一行) + AggregationNode::_merge_with_serialized_key->deserialize_and_merge_vec(執(zhí)行5次,每次merge一個分組) #取結(jié)果 _serialize_with_serialized_key_result-->serialize_to_column執(zhí)行一次,處理5個分組 _get_with_serialized_key_result-->insert_result_info5次,每次處理一個分組
group by + 一階段聚合
mysql>SELECTid,array_agg(label_name)FROMtest_array_aggGROUPBYid; +------+-------------------------+ |id|array_agg(`label_name`)| +------+-------------------------+ |1|["LC","LB","alex"]| |2|["LC","LB","LA"]| |3|["LC",NULL,"LA"]| |4|["LC","LB","LA"]| |5|["LC","LB","LA"]| +------+-------------------------+ 5rowsinset(20.12sec) #執(zhí)行 AggregationNode::_execute_with_serialized_key-->add(執(zhí)行15次,每次處理一行) #取結(jié)果 _get_with_serialized_key_result-->insert_result_info一次循環(huán),遍歷處理5個分組
group by + 多階段聚合
mysql>SELECTarray_agg(label_name)FROMtest_array_agg; +----------------------------------------------------------------------------------------------+ |array_agg(`label_name`)| +----------------------------------------------------------------------------------------------+ |["LC","LB","alex","LC","LB","LA","LC",NULL,"LA","LC","LB","LA","LC","LB","LA"]| +----------------------------------------------------------------------------------------------+ 1rowinset(1min21.01sec) #執(zhí)行 AggregationNode::_execute_without_key-->add(執(zhí)行15次,每次處理一行) AggregationNode::_merge_without_key-->deserialize_and_merge_from_column(執(zhí)行一次,只有一個分組,這個分組有15個元素) #取結(jié)果 AggregationNode::_serialize_without_key-->serialize_without_key_to_column AggregationNode::_get_without_key_result-->AggregateFunctionCollect::insert_result_into(執(zhí)行一次,只有一個分組,這個分組有15個元素)
03 函數(shù)調(diào)用棧
AggregationNode::init |-->//初始化_aggregate_evaluators |-->_aggregate_evaluators.reserve(tnode.agg_node.aggregate_functions.size()); |-->//beginloop |-->for(inti=0;i//為每個聚合函數(shù)生成一個evaluator |-->AggFnEvaluator::create(&evaluator) ||-->agg_fn_evaluator->_input_exprs_ctxs.push_back(ctx); |-->//將每個聚合函數(shù)的evaluator加到vector |-->_aggregate_evaluators.push_back(evaluator); |-->//endloop AggregationNode::prepare |-->ExecNode::prepare |-->AggregationNode::prepare_profile ||-->//beginloop ||-->for(inti=0;i//具體到某一個聚合函數(shù) ||-->_aggregate_evaluators[i]->prepare() |||-->//初始化groupby信息 |||-->VExpr::prepare() |||-->//初始化 |||-->AggFnEvaluator::prepare ||||-->//經(jīng)過一些工廠函數(shù)的處理,最終調(diào)用到具體的聚合函數(shù)的創(chuàng)建 ||||-->create_aggregate_function_collect |||||-->create_agg_function_map_agg(argument_types,result_is_nullable) ||||||-->//構(gòu)造函數(shù) ||||||-->AggregateFunctionCollect(constDataTypes&argument_types_) ||-->//endloop ||-->//bind各種函數(shù) //調(diào)用AggregationNode::sink函數(shù)對輸入block進(jìn)行聚合,該步驟會使用前面分配的execute函數(shù)進(jìn)行處理。 AggregationNode::sink |-->//in_block->rows()=15,就是數(shù)據(jù)的行數(shù) |-->_executor.execute(in_block) ||-->//groupby+分桶id(一階段聚合))即可觸發(fā) ||-->//SELECTid,array_agg(label_name)FROMtest_array_aggGROUPBYid; ||-->AggregationNode::_execute_with_serialized_key |||-->AggregationNode::_execute_with_serialized_key_helper ||||-->//這個時候num_rows就是所有記錄的行數(shù) ||||-->//但是這里循環(huán)了5次,因為有5個分組需要創(chuàng)建5個AggregateFunctionArrayAggData對象 ||||-->AggregationNode::_emplace_into_hash_table |||||-->PHHashMap ,false>::lazy_emplace_keys ||||||-->//開始遍歷所有的數(shù)據(jù)(keys.size()=15行) ||||||-->for(size_ti=0;i_hash_map.lazy_emplace_with_hash(keys[i],hash_values[i]..) |||||||-->//key不重復(fù)才會往下走,所以一共執(zhí)行了5次AggregateFunctionMapAggData |||||||-->creator-->AggregationNode::_create_agg_status ||||||||-->AggregationNode::_create_agg_status |||||||||-->AggFnEvaluator::create ||||||||||-->AggregateFunctionCollect::create |||||||||||-->//調(diào)用構(gòu)造函數(shù) |||||||||||-->AggregateFunctionArrayAggData() ||||||-->//結(jié)束遍歷 ||||-->//beginloop ||||-->for(inti=0;i//傳入block,此時block有15行 ||||-->_aggregate_evaluators[i]->execute_batch_add|AggFnEvaluator::execute_batch_add |||||-->//block->rows()=17offset=0_agg_columns.data()有兩列 |||||-->IAggregateFunctionHelper::add_batch |||||-->//beginloop |||||-->//batch_size=15,執(zhí)行15次add |||||-->for(size_ti=0;iAggregateFunctionCollect::add() |||||-->//endloop ||||-->//endloop || ||-->//不帶groupby+一階段聚合 ||-->AggregationNode::_execute_without_key |||-->AggFnEvaluator::execute_single_add ||||-->IAggregateFunctionHelper::add_batch_single_place ||||-->/*beginloop*/ ||||-->//執(zhí)行15次 ||||-->for(size_ti=0;iAggregateFunctionCollect::add() ||||-->//endloop ||-->//groupby+多階段聚合 ||-->AggregationNode::_merge_with_serialized_key |||-->AggregateFunctionCollect::deserialize_and_merge_vec ||-->//無groupby+多階段聚合 ||-->//SELECTarray_agg(label_name)FROMtest_array_agg; ||-->AggregationNode::_merge_without_key |||-->AggregateFunctionCollect::deserialize_and_merge_from_column ||-->AggregationNode::_pre_agg_with_serialized_key |||-->//如果聚合效果不佳,hash擴容達(dá)到閾值,則跳過聚合,直接把每一行輸入當(dāng)作一個分組 |||-->AggregateFunctionCollect::streaming_agg_serialize_to_column |||-->//如果hash擴容沒到閾值,還是采用樸素的方法 |||-->AggFnEvaluator::execute_batch_add ||||-->//執(zhí)行15次 ||||-->for(size_ti=0;iAggregateFunctionCollect::add() ||||-->//endloop AggregationNode::pull |-->//groupby+且需要finalize |-->//SELECTid,array_agg(label_name)FROMtest_array_aggGROUPBYid; |-->AggregationNode::_get_with_serialized_key_result ||-->AggregationNode::_get_result_with_serialized_key_non_spill |||-->//從block里面拿key的列,也就是groupby的列 |||-->key_columns.emplace_back |||-->//從block里面拿value的列 |||-->value_columns.emplace_back |||-->//如果是一階段聚合:這個時候num_rows=5,代表有5個分組 |||-->//SELECTid,array_agg(label_name)FROMtest_array_aggGROUPBYid; |||-->//如果是多階段聚合:這個時候num_rows=1,需要在上層調(diào)用5次 |||-->//SELECTlabel_name,array_agg(label_name)FROMtest_array_aggGROUPBYlabel_name; |||-->AggFnEvaluator::insert_result_info(num_rows) ||||-->for(size_ti=0;i!=num_rows;++i) ||||-->IAggregateFunctionHelper::insert_result_into |||||-->AggregateFunctionCollect::insert_result_into ||||||-->AggregateFunctionArrayAggData::insert_result_into ||||-->//循環(huán)結(jié)束 |-->//沒有g(shù)roupby且不需要finalize |-->AggregationNode::_serialize_without_key ||-->AggregateFunctionCollect::create_serialize_column ||-->AggregateFunctionCollect::serialize_without_key_to_column |||-->AggregateFunctionArrayAggData::insert_result_into |-->//沒有g(shù)roupby且需要finalize |-->AggregationNode::_get_without_key_result ||-->AggregateFunctionCollect::insert_result_into |||-->AggregateFunctionArrayAggData::insert_result_into |-->//groupby+且不需要finalize |-->AggregationNode::_serialize_with_serialized_key_result ||-->AggregationNode::_serialize_with_serialized_key_result_non_spill |||-->//num_rows=5,處理5個分組 |||-->AggregateFunctionCollect::serialize_to_column(num_rows) ||||-->AggregateFunctionArrayAggData::insert_result_into
注意點:
如果是兩階段聚合,在 execute 階段必然會執(zhí)行 execute+merge,即在會分別綁定 _merge_with 和 _execute_with,但是一階段聚合只會綁定 _execute_with;
如果是兩階段聚合,在 get_result 階段會有多個 AggregationNode,會根據(jù)具體的情況判斷是否 _needs_finalize;一階段聚合只有一個 AggregationNode,會綁定 _needs_finalize。
總結(jié)
最近由于工作需要筆者開始調(diào)研和使用 Apache Doris,通過閱讀聚合函數(shù)代碼切入 Apache Doris 內(nèi)核。秉承著開源的精神,開發(fā)了 array_agg 函數(shù)并貢獻(xiàn)給社區(qū)。希望通過這篇文章記錄下對源碼的一些理解,同時也方便后面的新人更快速地上手源碼開發(fā)。
在學(xué)習(xí)和掌握 Apache Doris 的過程中,作為 OLAP 新人的筆者遇到了很多疑惑點。好在 Apache Doris 不僅功能強大,社區(qū)更是十分活躍,社區(qū)技術(shù)大佬們對于新人的問題也特別熱心,不厭其煩幫我們新人們答疑解惑,這無疑為筆者在調(diào)研過程中增加了不少信心,在此由衷地感謝社區(qū)大佬 @yiguolei @mrhhsg。也期待未來有更多的小伙伴可以參與到社區(qū)當(dāng)中來,一同學(xué)習(xí)與成長。
作者介紹
隱形(邢穎) 網(wǎng)易資深數(shù)據(jù)庫內(nèi)核工程師,畢業(yè)至今一直從事數(shù)據(jù)庫內(nèi)核開發(fā)工作,目前主要參與 MySQL 與 Apache Doris 的開發(fā)維護(hù)和業(yè)務(wù)支持工作。
作為 MySQL 內(nèi)核貢獻(xiàn)者,為 MySQL 上報了 50 多個 Bug 及優(yōu)化項,多個提交被合入 MySQL 8.0 版本。從 2023 年起加入 Apache Doris 社區(qū),Apache Doris Active Contributor,已為社區(qū)提交并合入數(shù)十個 Commits。
審核編輯:湯梓紅
-
內(nèi)核
+關(guān)注
關(guān)注
3文章
1372瀏覽量
40275 -
數(shù)據(jù)庫
+關(guān)注
關(guān)注
7文章
3794瀏覽量
64358 -
源碼
+關(guān)注
關(guān)注
8文章
639瀏覽量
29185 -
函數(shù)
+關(guān)注
關(guān)注
3文章
4327瀏覽量
62569
原文標(biāo)題:Apache Doris 聚合函數(shù)源碼閱讀與解析
文章出處:【微信號:OSC開源社區(qū),微信公眾號:OSC開源社區(qū)】歡迎添加關(guān)注!文章轉(zhuǎn)載請注明出處。
發(fā)布評論請先 登錄
相關(guān)推薦
如何在ARMX64平臺上編譯Doris
OpenCV3編程入門-源碼例程全集-HoughLinesP函數(shù)
Navigation源碼解析
云海計費系統(tǒng)v4.1 視頻解析解析收費接口專用 短視頻解析解析收費接口專用 影視視頻電影解析計費平臺源碼程序
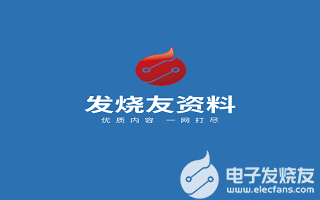
評論