在機(jī)器學(xué)習(xí)和數(shù)據(jù)科學(xué)的世界里,數(shù)據(jù)的質(zhì)量是建模成功與否的關(guān)鍵所在。這就是特征工程和數(shù)據(jù)預(yù)處理發(fā)揮作用的地方。本文總結(jié)的這些關(guān)鍵步驟可以顯著提高模型的性能,獲得更準(zhǔn)確的預(yù)測,我們將深入研究處理異常值、缺失值、編碼、特征縮放和特征提取的各種技術(shù)。
異常值異常值是數(shù)據(jù)集中與其他觀測值顯著不同的數(shù)據(jù)點(diǎn)。它們可能是由測量誤差、罕見事件或僅僅是數(shù)據(jù)自然變化的一部分引起的。識別和處理異常值是至關(guān)重要的,因為它們會扭曲統(tǒng)計分析并對模型性能產(chǎn)生負(fù)面影響。
有幾種方法可以檢測異常值:1、視覺方法:箱形圖、散點(diǎn)圖、直方圖2、統(tǒng)計方法:Z-score: Z-score > 3或< -3的點(diǎn)通常被認(rèn)為是異常值。四分位間距(IQR):低于Q1-1.5 * IQR或高于Q3 + 1.5 *IQR的數(shù)據(jù)點(diǎn)通常被視為異常值。3、機(jī)器學(xué)習(xí)方法:孤立森林、單類SVM、局部離群因子(LOF)而最常用的方法之一是使用四分位間距(IQR)方法
def outlier_thresholds(dataframe, col_name, q1=0.25, q3=0.75): quartile1 = dataframe[col_name].quantile(q1) quartile3 = dataframe[col_name].quantile(q3) interquantile_range = quartile3 - quartile1 up_limit = quartile3 + 1.5 * interquantile_range low_limit = quartile1 - 1.5 * interquantile_range return low_limit, up_limit
def check_outlier(dataframe, col_name): low_limit, up_limit = outlier_thresholds(dataframe, col_name) if dataframe[(dataframe[col_name] > up_limit) | (dataframe[col_name] < low_limit)].any(axis=None): return True else: return False
該函數(shù)計算IQR并將異常值定義為低于Q1-1.5 * IQR或高于Q3 + 1.5 * IQR的數(shù)據(jù)點(diǎn)。這個方法簡單快速,效果也很好。
異常值處理
1、刪除離群值
刪除異常值是一種直截了當(dāng)?shù)姆椒ǎ珣?yīng)該謹(jǐn)慎行事。只有在以下情況下才考慮刪除:
確定異常值是由于數(shù)據(jù)錯誤造成的。
數(shù)據(jù)集足夠大,刪除幾個點(diǎn)不會顯著影響你的分析。
- 異常值不能代表正在研究的人群。
刪除方法也很簡單:
def remove_outlier(dataframe, col_name): low_limit, up_limit = outlier_thresholds(dataframe, col_name) df_without_outliers = dataframe[~((dataframe[col_name] < low_limit) | (dataframe[col_name] > up_limit))] return df_without_outliers
2、帶閾值的重新分配可以將這些值限制在某個閾值,而不是刪除。這種方法也被稱為winsorization。以下是使用threshold重新賦值的代碼示例:
def replace_with_thresholds(dataframe, variable): low_limit, up_limit = outlier_thresholds(dataframe, variable) dataframe.loc[(dataframe[variable] < low_limit), variable] = low_limit dataframe.loc[(dataframe[variable] > up_limit), variable] = up_limit
多元離群分析:局部離群因子
LOF算法:圖像中的A點(diǎn)比其鄰近點(diǎn)的密度更稀疏,距離更遠(yuǎn)。在這種情況下,可以說點(diǎn)A是一個異常值。
LOF是一種通過測量數(shù)據(jù)點(diǎn)相對于其鄰居的局部偏差來識別異常值的算法。LOF將一個點(diǎn)的局部密度與其相鄰點(diǎn)的局部密度進(jìn)行比較,從而識別出密度明顯低于相鄰點(diǎn)的樣本。以下是多元離群分析的代碼示例:
from sklearn.neighbors import LocalOutlierFactor
def detect_outliers_lof(data, n_neighbors=20): lof = LocalOutlierFactor(n_neighbors=n_neighbors, contamination='auto') outlier_labels = lof.fit_predict(data) return outlier_labels == -1 # True for outliers, False for inliers
缺失值缺失值是現(xiàn)實世界數(shù)據(jù)集中常見的問題,處理丟失數(shù)據(jù)時要考慮的一個重要問題是丟失數(shù)據(jù)的隨機(jī)性。在Python中,你可以使用pandas輕松檢測缺失值:
def missing_values_table(dataframe, na_name=False): na_columns = [col for col in dataframe.columns if dataframe[col].isnull().sum() > 0]
n_miss = dataframe[na_columns].isnull().sum().sort_values(ascending=False) ratio = (dataframe[na_columns].isnull().sum() / dataframe.shape[0] * 100).sort_values(ascending=False) missing_df = pd.concat([n_miss, np.round(ratio, 2)], axis=1, keys=['n_miss', 'ratio']) print(missing_df, end="\n")
if na_name: return na_columns
缺失值處理
1、刪除缺失值:如果缺失值的數(shù)量相對于數(shù)據(jù)集大小較小,則刪除可能是一種有效的策略。
def remove_missing(df, threshold=0.7): return df.dropna(thresh=int(threshold*len(df)), axis=1).dropna()
2、用簡單的方法填充簡單的插值方法包括用均值、中位數(shù)或眾數(shù)填充:
def simple_impute(dataframe):
cat_cols = [col for col in dataframe.columns if dataframe[col].dtypes == "O"] num_but_cat = [col for col in dataframe.columns if dataframe[col].nunique() < cat_th and dataframe[col].dtypes != "O"] cat_but_car = [col for col in dataframe.columns if dataframe[col].nunique() > car_th and dataframe[col].dtypes == "O"] cat_cols = cat_cols + num_but_cat cat_cols = [col for col in cat_cols if col not in cat_but_car]
num_cols = [col for col in dataframe.columns if dataframe[col].dtypes != "O"] num_cols = [col for col in num_cols if col not in num_but_cat]
df[num_cols] = df[num_cols].fillna(df[num_cols].median()) df[cat_cols] = df[cat_cols].fillna(df[cat_cols].mode().iloc[0])
return df
3、分類變量分解中的值對于數(shù)值變量,可以根據(jù)相關(guān)分類變量的平均值或中位數(shù)填充缺失值:
def categorical_impute(df, col_1, col_2, method="mean"): df[col_1].fillna(df.groupby(col_2)[col_1].transform(method)) return df
4、預(yù)測賦值填充KNN Imputer (K-Nearest Neighbors Imputer)是一種處理數(shù)據(jù)集中缺失數(shù)據(jù)的方法:它基于k近鄰算法。對于每個缺失值的樣本,它找到K個最相似的完整樣本。然后使用這些鄰居的值來估計和填充缺失的數(shù)據(jù)。輸入值通常是相鄰值的平均值或中值。當(dāng)丟失的數(shù)據(jù)不是隨機(jī)的并且依賴于其他特征時,它特別有用。KNN Imputer比mean或median imputation等簡單的imputation方法更準(zhǔn)確,特別是對于特征之間的關(guān)系很重要的數(shù)據(jù)集。但是對于大型數(shù)據(jù)集來說,它的計算成本很高。
from sklearn.impute import KNNImputer
def knn_impute(dataframe, n_neighbors=5):
cat_cols = [col for col in dataframe.columns if dataframe[col].dtypes == "O"] num_but_cat = [col for col in dataframe.columns if dataframe[col].nunique() < cat_th and dataframe[col].dtypes != "O"] cat_but_car = [col for col in dataframe.columns if dataframe[col].nunique() > car_th and dataframe[col].dtypes == "O"] cat_cols = cat_cols + num_but_cat cat_cols = [col for col in cat_cols if col not in cat_but_car]
num_cols = [col for col in dataframe.columns if dataframe[col].dtypes != "O"] num_cols = [col for col in num_cols if col not in num_but_cat]
df = pd.get_dummies(dataframe[cat_cols + num_cols], drop_first=True)
# Standardization of Variables scaler = MinMaxScaler() df = pd.DataFrame(scaler.fit_transform(df), columns=df.columns) df.head()
# Implementation of KNN
imputer = KNNImputer(n_neighbors=n_neighbors)
return pd.DataFrame(imputer.fit_transform(df), columns=df.columns)
編碼
編碼是將分類變量轉(zhuǎn)換為可以提供給機(jī)器學(xué)習(xí)算法使用的格式的過程。一般包括標(biāo)簽編碼:為類別分配唯一的數(shù)字標(biāo)簽。獨(dú)熱編碼:將分類變量轉(zhuǎn)換為二進(jìn)制向量。稀有編碼:當(dāng)一個分類變量有一些在數(shù)據(jù)集中很少出現(xiàn)的類別時,使用這種技術(shù)。這些編碼有助于將各種數(shù)據(jù)類型轉(zhuǎn)換為數(shù)字格式,使機(jī)器學(xué)習(xí)模型能夠提取模式并更準(zhǔn)確地進(jìn)行預(yù)測。標(biāo)簽編碼:標(biāo)簽編碼用于將分類數(shù)據(jù)轉(zhuǎn)換為算法可以處理的數(shù)字格式。它的工作原理是為分類變量中的每個類別分配一個唯一的整數(shù)。此方法對于類別有自然順序的有序數(shù)據(jù)特別有用,例如評級。但是標(biāo)簽編碼可能會在不存在的類別之間引入人為的順序關(guān)系,這對于某些算法來說可能是有問題的。
from sklearn.preprocessing import LabelEncoder
def label_encoder(dataframe, binary_col): labelencoder = LabelEncoder() dataframe[binary_col] = labelencoder.fit_transform(dataframe[binary_col]) return dataframe
binary_cols = [col for col in df.columns if df[col].dtype not in [int, float] and df[col].nunique() == 2]
for col in binary_cols: label_encoder(df, col)
獨(dú)熱編碼:獨(dú)熱編碼是一種用于數(shù)字表示分類數(shù)據(jù)的技術(shù),適用于需要數(shù)字輸入的機(jī)器學(xué)習(xí)算法。在這種方法中,特征中的每個唯一類別成為一個新的二進(jìn)制列。對于給定的類別,相應(yīng)的列被設(shè)置為1(或“hot”),而所有其他列都被設(shè)置為0。這種方法允許在不暗示類別之間的任何順序關(guān)系的情況下表示類別變量。它在處理標(biāo)稱數(shù)據(jù)時特別有用,因為類別沒有固有的順序或?qū)哟谓Y(jié)構(gòu)。但是如果分類數(shù)據(jù)中的類別較多會增加稀疏性。
def one_hot_encoder(dataframe, categorical_cols, drop_first=True): dataframe = pd.get_dummies(dataframe, columns=categorical_cols, drop_first=drop_first) return dataframe
ohe_cols = [col for col in df.columns if 10 >= df[col].nunique() > 2]
one_hot_encoder(df, ohe_cols).head()
稀有編碼:機(jī)器學(xué)習(xí)中的稀有編碼通常是指用于處理分類變量中罕見或不常見類別的技術(shù)。當(dāng)一個分類變量有一些在數(shù)據(jù)集中很少出現(xiàn)的類別時,使用這種技術(shù)可以防止過擬合,降低這些罕見類別給模型帶來的噪聲。
- 將不常見的類別分組:將不常見的類別合并到一個“其他”類別中。
- 基于頻率的編碼:用數(shù)據(jù)集中的頻率替換稀有類別。
- 基于相似性的編碼:根據(jù)與更常見的類別的相似性對罕見類別進(jìn)行分組。
設(shè)置頻率閾值(例如,少于1%的出現(xiàn))來定義什么構(gòu)成“罕見”類別。這樣有助于降低模型的復(fù)雜性,改進(jìn)泛化,并處理測試數(shù)據(jù)中未見過的類別。
cat_cols = [col for col in dataframe.columns if dataframe[col].dtypes == "O"] num_but_cat = [col for col in dataframe.columns if dataframe[col].nunique() < cat_th and dataframe[col].dtypes != "O"] cat_but_car = [col for col in dataframe.columns if dataframe[col].nunique() > car_th and dataframe[col].dtypes == "O"] cat_cols = cat_cols + num_but_cat cat_cols = [col for col in cat_cols if col not in cat_but_car]
def rare_analyser(dataframe, target, cat_cols): for col in cat_cols: print(col, ":", len(dataframe[col].value_counts())) print(pd.DataFrame({"COUNT": dataframe[col].value_counts(), "RATIO": dataframe[col].value_counts() / len(dataframe), "TARGET_MEAN": dataframe.groupby(col)[target].mean()}), end="\n\n\n")
rare_analyser(df, "TARGET", cat_cols)
def rare_encoder(dataframe, rare_perc): temp_df = dataframe.copy()
rare_columns = [col for col in temp_df.columns if temp_df[col].dtypes == 'O' and (temp_df[col].value_counts() / len(temp_df) < rare_perc).any(axis=None)]
for var in rare_columns: tmp = temp_df[var].value_counts() / len(temp_df) rare_labels = tmp[tmp < rare_perc].index temp_df[var] = np.where(temp_df[var].isin(rare_labels), 'Rare', temp_df[var])
return temp_df
new_df = rare_encoder(df, 0.01)
特征縮放
特征縮放是一種用于機(jī)器學(xué)習(xí)的預(yù)處理技術(shù),用于標(biāo)準(zhǔn)化數(shù)據(jù)的自變量或特征的范圍。因為特征在相同條件下可以減少算法的訓(xùn)練時間。當(dāng)變量被標(biāo)準(zhǔn)化時,減少由縮放特征產(chǎn)生的誤差的努力會更容易。因為在同一條件下可以確保所有特征對模型的性能貢獻(xiàn)相同,防止較大的特征主導(dǎo)學(xué)習(xí)過程。這對輸入特征的尺度敏感的算法尤其重要,例如基于梯度下降的算法和基于距離的算法。當(dāng)特征處于相似規(guī)模時,許多機(jī)器學(xué)習(xí)算法表現(xiàn)更好或收斂更快。但是應(yīng)分別應(yīng)用于訓(xùn)練集和測試集,以避免數(shù)據(jù)泄漏。
Standard Scaling標(biāo)準(zhǔn)化對特征進(jìn)行縮放,使它們的均值為0,方差為1。
from sklearn.preprocessing import StandardScaler
def standard_scale(df, columns): scaler = StandardScaler() df[columns] = scaler.fit_transform(df[columns]) return df
Robust ScalingRobust Scaling使用對異常值具有魯棒性的統(tǒng)計信息。
from sklearn.preprocessing import RobustScaler
def robust_scale(df, columns): scaler = RobustScaler() df[columns] = scaler.fit_transform(df[columns]) return df
Min-Max ScalingMinMax Scaling將特征縮放到一個固定的范圍,通常在0到1之間。
from sklearn.preprocessing import MinMaxScaler
def minmax_scale(df, columns): scaler = MinMaxScaler() df[columns] = scaler.fit_transform(df[columns]) return df
分箱分箱是通過創(chuàng)建一組區(qū)間將連續(xù)變量轉(zhuǎn)換為分類變量的過程。
import numpy as np
def binning(df, column, bins, labels=None): df[f'{column}_binned'] = pd.qcut(df[column], bins=bins, labels=labels) return df
特征提取
特征提取是機(jī)器學(xué)習(xí)和數(shù)據(jù)分析中的一項重要技術(shù)。它包括選擇原始數(shù)據(jù)并將其轉(zhuǎn)換為一組更有用的特征,這些特征可用于進(jìn)一步處理或分析。特征提取的目的是,降低數(shù)據(jù)的維數(shù),這樣可以簡化模型,提高性能。文本統(tǒng)計特征創(chuàng)建二進(jìn)制特征可以突出顯示數(shù)據(jù)中的重要特征。
def create_binary_feature(df, column, condition): df[f'{column}_flag'] = np.where(condition(df[column]), 1, 0) return df
例如對于下面的文本文本數(shù)據(jù)通常包含有價值的信息,這些信息可以提取為數(shù)字特征。
# Letter Count
df["NEW_NAME_COUNT"] = df["Name"].str.len()
# Word Count
df["NEW_NAME_WORD_COUNT"] = df["Name"].apply(lambda x: len(str(x).split(" ")))
# Capturing Special Structures
df["NEW_NAME_DR"] = df["Name"].apply(lambda x: len([x for x in x.split() if x.startswith("Dr")]))
df.groupby("NEW_NAME_DR").agg({"Survived": ["mean","count"]})
# Deriving Variables with Regex
df['NEW_TITLE'] = df.Name.str.extract(' ([A-Za-z]+)\.', expand=False)
df[["NEW_TITLE", "Survived", "AGE"]].groupby(["NEW_TITLE"]).agg({"Survived": "mean", "AGE": ["count", "mean"]})
時間序列變量對于時間序列可以將日期變量分解為與分析相關(guān)的各種子組件。
def date_features(df, date_column): df[f'{date_column}_year'] = df[date_column].dt.year df[f'{date_column}_month'] = df[date_column].dt.month df[f'{date_column}_day'] = df[date_column].dt.day df[f'{date_column}_dayofweek'] = df[date_column].dt.dayofweek return df
這樣就可以針對不同的時間進(jìn)行處理。
總結(jié)特征工程和數(shù)據(jù)預(yù)處理是任何機(jī)器學(xué)習(xí)中的關(guān)鍵步驟。它們可以通過確保數(shù)據(jù)干凈、結(jié)構(gòu)良好和信息豐富來顯著提高模型的性能。本文介紹了如何處理異常值和缺失值、編碼分類變量、縮放數(shù)值特征和創(chuàng)建新特征——為準(zhǔn)備機(jī)器學(xué)習(xí)任務(wù)的數(shù)據(jù)奠定了堅實的基礎(chǔ)。
我們這里也只是介紹一些簡單常見的技術(shù),使用更復(fù)雜和更具體技術(shù)將取決于數(shù)據(jù)集和試圖解決的問題。
作者:Kursat Dinc
本文來源:DeepHub IMBA
-
檢測
+關(guān)注
關(guān)注
5文章
4480瀏覽量
91442 -
機(jī)器學(xué)習(xí)
+關(guān)注
關(guān)注
66文章
8406瀏覽量
132561 -
數(shù)據(jù)預(yù)處理
+關(guān)注
關(guān)注
1文章
20瀏覽量
2756
發(fā)布評論請先 登錄
相關(guān)推薦
Python數(shù)據(jù)預(yù)處理方法
數(shù)據(jù)探索與數(shù)據(jù)預(yù)處理
數(shù)據(jù)預(yù)處理故障信息獲取
工業(yè)蒸汽量預(yù)測的數(shù)據(jù)預(yù)處理知識有哪些
全志R128芯片 如何在FreeRTOS下對代碼源文件進(jìn)行快速預(yù)處理?
dvteclipse代碼預(yù)處理的簡單操作方法推薦
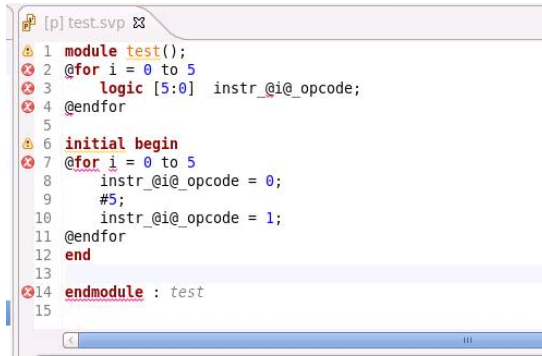
機(jī)器學(xué)習(xí)的特征預(yù)處理問題討論
C語言-預(yù)處理(#define、#if...)
機(jī)器學(xué)習(xí)算法學(xué)習(xí)之特征工程1
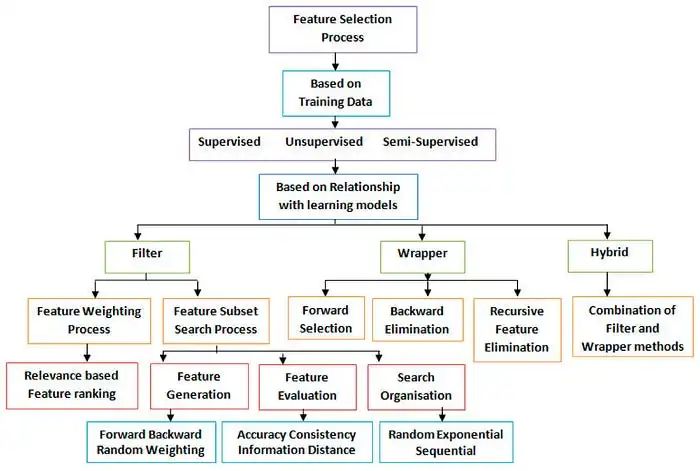
機(jī)器學(xué)習(xí)算法學(xué)習(xí)之特征工程2
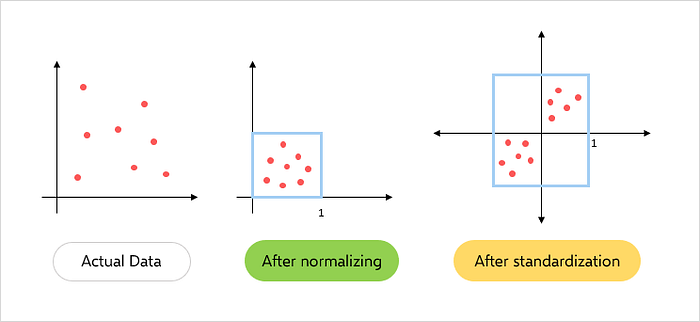
機(jī)器學(xué)習(xí)算法學(xué)習(xí)之特征工程3
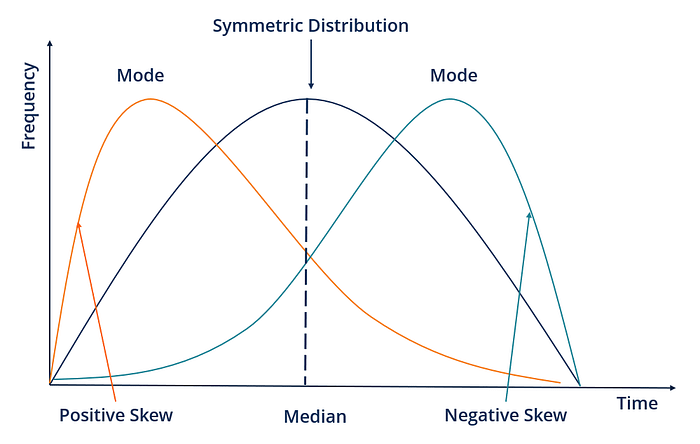
C語言有哪些預(yù)處理操作?
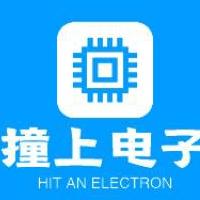
評論