導讀 本文主要講解如何將pytorch的模型部署到c++平臺上的模型流程,按順序分為四大塊詳細說明了模型轉換、保存序列化模型、C ++中加載序列化的PyTorch模型以及執(zhí)行Script Module。
最近因為工作需要,要把pytorch的模型部署到c++平臺上,基本過程主要參照官網的教學示例,期間發(fā)現了不少坑,特此記錄。
1.模型轉換
libtorch不依賴于python,python訓練的模型,需要轉換為script model才能由libtorch加載,并進行推理。在這一步官網提供了兩種方法: 方法一:Tracing 這種方法操作比較簡單,只需要給模型一組輸入,走一遍推理網絡,然后由torch.ji.trace記錄一下路徑上的信息并保存即可。示例如下:
importtorch importtorchvision #Aninstanceofyourmodel. model=torchvision.models.resnet18() #Anexampleinputyouwouldnormallyprovidetoyourmodel'sforward()method. example=torch.rand(1,3,224,224) #Usetorch.jit.tracetogenerateatorch.jit.ScriptModuleviatracing. traced_script_module=torch.jit.trace(model,example) 缺點是如果模型中存在控制流比如if-else語句,一組輸入只能遍歷一個分支,這種情況下就沒辦法完整的把模型信息記錄下來。 方法二:Scripting 直接在Torch腳本中編寫模型并相應地注釋模型,通過torch.jit.script編譯模塊,將其轉換為ScriptModule。示例如下:
classMyModule(torch.nn.Module): def__init__(self,N,M): super(MyModule,self).__init__() self.weight=torch.nn.Parameter(torch.rand(N,M)) defforward(self,input): ifinput.sum()>0: output=self.weight.mv(input) else: output=self.weight+input returnoutput my_module=MyModule(10,20) sm=torch.jit.script(my_module)
forward方法會被默認編譯,forward中被調用的方法也會按照被調用的順序被編譯
如果想要編譯一個forward以外且未被forward調用的方法,可以添加@torch.jit.export.
如果想要方法不被編譯,可使用[@torch.jit.ignore](https://link.zhihu.com/?target=https%3A//pytorch.org/docs/master/generated/torch.jit.ignore.html%23torch.jit.ignore)或者[@torch.jit.unused](https://link.zhihu.com/?target=https%3A//pytorch.org/docs/master/generated/torch.jit.unused.html%23torch.jit.unused)
#Samebehavioraspre-PyTorch1.2 @torch.jit.script defsome_fn(): return2 #Marksafunctionasignored,ifnothing #evercallsitthenthishasnoeffect @torch.jit.ignore defsome_fn2(): return2 #Aswithignore,ifnothingcallsitthenithasnoeffect. #Ifitiscalledinscriptitisreplacedwithanexception. @torch.jit.unused defsome_fn3(): importpdb;pdb.set_trace() return4 #Doesn'tdoanything,thisfunctionisalready #themainentrypoint @torch.jit.export defsome_fn4(): return2 在這一步遇到好多坑,主要原因可歸為一下兩點
1. 不支持的操作
TorchScript支持的操作是python的子集,大部分torch中用到的操作都可以找到對應實現,但也存在一些尷尬的不支持操作,詳細列表可見unsupported-ops(https://pytorch.org/docs/master/jit_unsupported.html#jit-unsupported),下面列一些我自己遇到的操作: 1)參數/返回值不支持可變個數,例如
def__init__(self,**kwargs): 或者
ifoutput_flag==0: returnreshape_logits else: loss=self.loss(reshape_logits,term_mask,labels_id) returnreshape_logits,loss 2)各種iteration操作 eg1.
layers=[int(a)forainlayers] 報錯torch.jit.frontend.UnsupportedNodeError: ListComp aren’t supported 可以改成:
forkinrange(len(layers)): layers[k]=int(layers[k]) eg2.
seq_iter=enumerate(scores) try: _,inivalues=seq_iter.__next__() except: _,inivalues=seq_iter.next() eg3.
line=next(infile) 3)不支持的語句 eg1. 不支持continue torch.jit.frontend.UnsupportedNodeError: continue statements aren’t supported eg2. 不支持try-catch torch.jit.frontend.UnsupportedNodeError: try blocks aren’t supported eg3. 不支持with語句 4)其他常見op/module eg1. torch.autograd.Variable 解決:使用torch.ones/torch.randn等初始化+.float()/.long()等指定數據類型。 eg2. torch.Tensor/torch.LongTensor etc. 解決:同上 eg3. requires_grad參數只在torch.tensor中支持,torch.ones/torch.zeros等不可用 eg4. tensor.numpy() eg5. tensor.bool() 解決:tensor.bool()用tensor>0代替 eg6. self.seg_emb(seg_fea_ids).to(embeds.device) 解決:需要轉gpu的地方顯示調用.cuda() 總之一句話:除了原生python和pytorch以外的庫,比如numpy什么的能不用就不用,盡量用pytorch的各種API。
2. 指定數據類型
1)屬性,大部分的成員數據類型可以根據值來推斷,空的列表/字典則需要預先指定
fromtypingimportDict classMyModule(torch.nn.Module): my_dict:Dict[str,int] def__init__(self): super(MyModule,self).__init__() #Thistypecannotbeinferredandmustbespecified self.my_dict={} #Theattributetypehereisinferredtobe`int` self.my_int=20 defforward(self): pass m=torch.jit.script(MyModule()) 2)常量,使用_Final_關鍵字
try: fromtyping_extensionsimportFinal except: #Ifyoudon'thave`typing_extensions`installed,youcanusea #polyfillfrom`torch.jit`. fromtorch.jitimportFinal classMyModule(torch.nn.Module): my_constant:Final[int] def__init__(self): super(MyModule,self).__init__() self.my_constant=2 defforward(self): pass m=torch.jit.script(MyModule()) 3)變量。默認是tensor類型且不可變,所以非tensor類型必須要指明
defforward(self,batch_size:int,seq_len:int,use_cuda:bool): 方法三:Tracing and Scriptin混合 一種是在trace模型中調用script,適合模型中只有一小部分需要用到控制流的情況,使用實例如下:
importtorch @torch.jit.script deffoo(x,y): ifx.max()>y.max(): r=x else: r=y returnr defbar(x,y,z): returnfoo(x,y)+z traced_bar=torch.jit.trace(bar,(torch.rand(3),torch.rand(3),torch.rand(3))) 另一種情況是在script module中用tracing生成子模塊,對于一些存在script module不支持的python feature的layer,就可以把相關layer封裝起來,用trace記錄相關layer流,其他layer不用修改。使用示例如下:
importtorch importtorchvision classMyScriptModule(torch.nn.Module): def__init__(self): super(MyScriptModule,self).__init__() self.means=torch.nn.Parameter(torch.tensor([103.939,116.779,123.68]) .resize_(1,3,1,1)) self.resnet=torch.jit.trace(torchvision.models.resnet18(), torch.rand(1,3,224,224)) defforward(self,input): returnself.resnet(input-self.means) my_script_module=torch.jit.script(MyScriptModule())
2.保存序列化模型
如果上一步的坑都踩完,那么模型保存就非常簡單了,只需要調用save并傳遞一個文件名即可,需要注意的是如果想要在gpu上訓練模型,在cpu上做inference,一定要在模型save之前轉化,再就是記得調用model.eval(),形如
gpu_model.eval() cpu_model=gpu_model.cpu() sample_input_cpu=sample_input_gpu.cpu() traced_cpu=torch.jit.trace(traced_cpu,sample_input_cpu) torch.jit.save(traced_cpu,"cpu.pth") traced_gpu=torch.jit.trace(traced_gpu,sample_input_gpu) torch.jit.save(traced_gpu,"gpu.pth")
3.C++ load訓練好的模型
要在C ++中加載序列化的PyTorch模型,必須依賴于PyTorch C ++ API(也稱為LibTorch)。libtorch的安裝非常簡單,只需要在pytorch官網下載對應版本,解壓即可。會得到一個結構如下的文件夾。
libtorch/ bin/ include/ lib/ share/ 然后就可以構建應用程序了,一個簡單的示例目錄結構如下:
example-app/ CMakeLists.txt example-app.cpp example-app.cpp和CMakeLists.txt的示例代碼分別如下:
#include
cmake_minimum_required(VERSION 3.0 FATAL_ERROR) project(custom_ops) find_package(Torch REQUIRED) add_executable(example-app example-app.cpp) target_link_libraries(example-app "${TORCH_LIBRARIES}") set_property(TARGET example-app PROPERTY CXX_STANDARD 14) 至此,就可以運行以下命令從example-app/文件夾中構建應用程序啦:
mkdir build cd build cmake -DCMAKE_PREFIX_PATH=/path/to/libtorch .. cmake --build . --config Release 其中/path/to/libtorch是之前下載后的libtorch文件夾所在的路徑。這一步如果順利能夠看到編譯完成100%的提示,下一步運行編譯生成的可執(zhí)行文件,會看到“ok”的輸出,可喜可賀!
4. 執(zhí)行Script Module
終于到最后一步啦!下面只需要按照構建輸入傳給模型,執(zhí)行forward就可以得到輸出啦。一個簡單的示例如下:
//Createavectorofinputs. std::vector
-
C++
+關注
關注
22文章
2108瀏覽量
73618 -
模型
+關注
關注
1文章
3226瀏覽量
48806 -
pytorch
+關注
關注
2文章
807瀏覽量
13198
原文標題:C++平臺PyTorch模型部署流程,踩坑心得實錄
文章出處:【微信號:vision263com,微信公眾號:新機器視覺】歡迎添加關注!文章轉載請注明出處。
發(fā)布評論請先 登錄
相關推薦
如何使用OpenVINO C++ API部署FastSAM模型
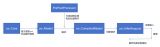
如何將AI模型部署到嵌入式系統(tǒng)中
將pytorch模型轉化為onxx模型的步驟有哪些
通過Cortex來非常方便的部署PyTorch模型
將Pytorch模型轉換為DeepViewRT模型時出錯怎么解決?
如何使用TensorFlow將神經網絡模型部署到移動或嵌入式設備上
pytorch模型轉換需要注意的事項有哪些?
使用Cortex將PyTorch模型部署到生產中
如何將Pytorch自訓練模型變成OpenVINO IR模型形式
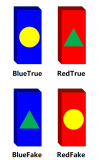
TorchVision框架下模型導出并部署到ONNXRUNTIME C++全流程解析
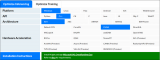
基于OpenVINO C++ API部署RT-DETR模型
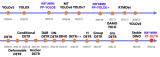
評論